
The quantitative and qualitative evaluation of a multi-agent microsimulation model for subway carriage design
Cite this article
as: L. Cao, X. Li, F. Kang, C. Liu, F. Sun, R. Kotagiri; 2015; The quantitative and qualitative evaluation of a multi-agent microsimulation model for subway carriage design; International Journal of Microsimulation; 8(3); 6-40.
doi: 10.34196/ijm.00120
- Article
- Figures and data
- Jump to
Figures
Figure 6
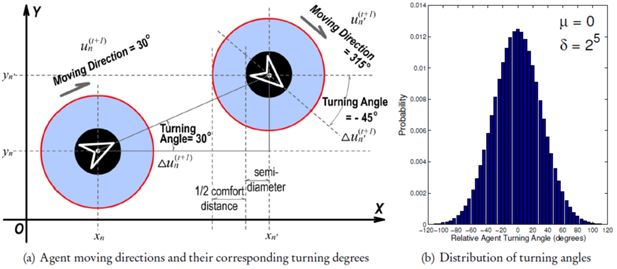
Illustration of agent turning angle that is selected obeying a discrete Gaussian distribution.
Figure 8
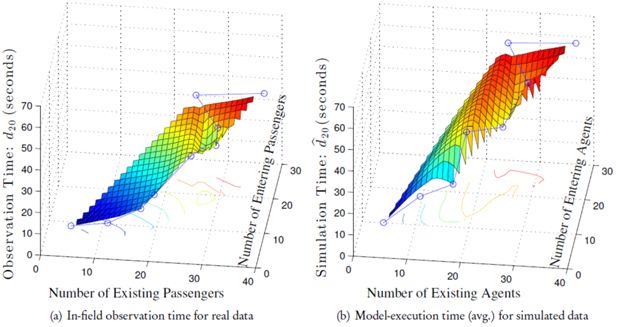
The observation/simulation time (blue line) and the corresponding cubic interpolations (3D surface) as a function of existing and entering passengers/agents.
Figure 9
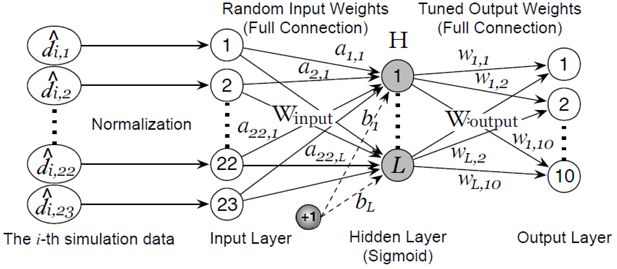
The structure of a typical ELM network with one hidden layer (with L nodes), one input layer (with 23 nodes), and one output layer (with 10 nodes representing 10 categories).
Figure 10
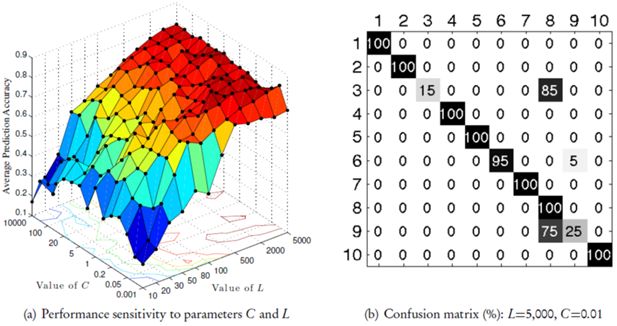
The evaluation results obtained via an unified multinomial classifier: (a) the performance sensitivity obtained via grid searching; (b) the class-wise confusion matrix of prediction accuracy.
Tables
Table 1
Real data collected from in-field observation.
di | Initial Status (entering|existing passengers) | Real-data Dimensions (i.e. di,1, di,2,…, di,23) |
d1 | 17-01,19-03|16:1,17:2,18:1,19:1 | 1,0,0,0,0,0,0,0,0,0,0,0,0,1,1,2,1,2,1,08,0,1,0 |
d2 | 17-03,19-00|14:1,16:3,17:2,18:2,19:4 | 0,1,0,0,0,0,0,0,0,0,0,0,0,2,1,1,2,4,4,12,4,3,0 |
d3 | 16-01,18-03|9:1,14:3,15:3,16:4,17:3,18:2,19:2 | 1,1,1,0,0,0,0,0,1,0,0,0,0,3,3,2,4,3,3,18,1,1,0 |
d4 | 16-02,18-05|1:1,14:3,15:3,16:4,17:2,18:4,19:3 | 3,2,3,0,0,0,0,0,0,0,0,0,0,4,4,3,3,2,3,20,3,1,1 |
d5 | 16-07,18-05|1:1,2:2,14:3,15:2,16:5,17:5,18:5,19:3 | 4,2,3,0,0,1,0,0,0,0,0,0,0,5,7,4,2,6,4,31,6,3,2 |
d6 | 17-09,19-08|1:2,2:4,14:2,15:2,16:7,17:3,18:4,19:4 | 3,3,2,0,0,1,1,0,0,0,1,0,0,6,7,6,5,4,6,28,3,1,1 |
d7 | 16-10,18-06|1:4,3:1,4:1,7:1,14:4,15:2,16:5,17:4,18:4,19:4 | 3,2,2,0,0,0,1,0,0,0,0,0,0,5,9,7,6,4,7,30,7,4,3 |
d8 | 17-08,19-10|1:2,2:2,3:2,4:1,8:1,14:2,15:2,16:5,17:2,18:6,19:5 | 4,4,3,1,0,0,0,1,0,0,0,0,0,5,8,3,8,7,4,35,0,3,5 |
d9 | 17-11,19-13|1:2,2:2,3:1,6:1,8:1,14:3,15:3,16:4,17:3,18:2,19:3 | 2,4,2,0,1,0,0,0,1,1,0,0,0,6,8,6,5,5,8,40,3,3,4 |
d10 | 16-10,18-11|1:3,2:4,3:2,6:1,7:1,10:1,14:5,15:4,16:5,17:4,18:4,19:5 | 4,6,3,0,0,1,1,1,0,1,0,0,0,8,9,8,7,5,6,46,4,6,5 |
Table 2
Partial simulation data samples generated from 100 valid micro-model executions.
Model Initialization (entering|existing agents) | Features of Simulation Data | |
17–01,19–03|16:1,17:2,18:1,19:1 | 1,0,0,0,0,0,0,0,0,0,0,0,0,0,0,4,0,3,1,05,00,1,1 | |
17–01,19–03|16:1,17:2,18:1,19:1 | 1,0,1,1,0,0,0,0,0,0,0,0,0,1,0,1,1,0,3,10,00,0,4 | |
17–01,19–03|16:1,17:2,18:1,19:1 | 1,0,1,0,0,0,0,0,0,0,0,0,0,1,0,3,1,1,1,13,00,2,3 | |
17–01,19–03|16:1,17:2,18:1,19:1 | 1,0,3,1,0,0,0,0,0,0,0,0,0,0,0,1,1,1,1,18,04,4,4 | |
17–01,19–03|16:1,17:2,18:1,19:1 | 1,0,2,1,0,0,0,0,0,0,0,0,0,1,0,1,1,1,1,10,02,3,5 | |
17–01,19–03|16:1,17:2,18:1,19:1 | 1,0,3,1,0,0,0,0,0,0,0,0,0,0,0,1,1,1,1,09,03,3,4 | |
17–01,19–03|16:1,17:2,18:1,19:1 | 1,0,3,1,0,0,0,0,0,0,0,0,0,0,0,1,1,1,1,10,02,2,4 | |
17–01,19–03|16:1,17:2,18:1,19:1 | 1,0,1,1,0,0,0,0,0,0,0,0,0,0,0,1,1,1,3,08,01,1,3 | |
17–01,19–03|16:1,17:2,18:1,19:1 | 1,0,0,0,0,0,0,0,0,0,0,0,0,0,0,3,1,3,1,05,00,1,2 | |
17–01,19–03|16:1,17:2,18:1,19:1 | 1,0,0,0,0,0,0,0,0,0,0,0,0,0,0,4,0,1,3,07,00,1,1 | |
17–03,19–00|14:1,16:3,17:2,18:2,19:4 | 1,0,1,0,1,0,0,0,0,0,0,0,1,0,0,3,4,2,2,23,05,5,0 | |
16–01,18–03|9:1,14:3,15:3,16:4,17:3,18:2,19:2 | 1,1,1,0,1,0,0,0,0,0,0,0,1,1,2,5,1,4,4,27,02,3,1 | |
16–02,18–05|1:1,14:3,15:3,16:4,17:2,18:4,19:3 | 3,2,2,0,0,1,0,0,0,0,0,1,0,4,4,2,3,2,3,43,05,1,1 | |
16–07,18–05|1:1,2:2,14:3,15:2,16:5,17:5,18:5,19:3 | 3,3,2,0,0,1,0,0,1,0,1,0,1,5,5,4,3,6,3,46,07,2,2 | |
17–09,19–08|1:2,2:4,14:2,15:2,16:7,17:3,18:4,19:4 | 4,2,3,0,1,2,0,0,0,1,0,0,0,6,5,7,5,6,3,47,05,2,1 | |
16–10,18–06|1:4,3:1,4:1,7:1,14:4,15:2,16:5,17:4,18:4,19:4 | 3,1,2,1,0,1,0,1,0,1,0,0,0,4,7,8,6,5,6,52,10,3,4 | |
17–08,19–10|1:2,2:2,3:2,4:1,8:1,14:2,15:2,16:5,17:2,18:6,19:5 | 3,5,3,0,2,0,1,0,0,1,0,0,1,5,6,4,6,6,5,56,01,3,5 | |
17–11,19–13|1:2,2:2,3:1,6:1,8:1,14:3,15:3,16:4,17:3,18:2,19:3 | 2,5,3,0,0,1,1,0,1,0,0,1,1,7,5,6,5,5,6,63,04,2,4 | |
16–10,18–111:3,2:4,3:2,6:1,7:1,10:1,14:5,15:4,16:5,17:4,18:4,19:5 | 5,6,2,0,2,1,1,0,1,0,1,0,1,7,7,8,6,6,6,69,04,5,5 |
Table 3
Parameters and measures of 10 one-vs.-rest binary classifiers (200 trials); the scores labeled with bold, asterisk, and underline represent the best, 2nd best, and the worst results respectively.
Parameters & Measures | Class. k=1 | Class. k=2 | Class. k=3 | Class. k=4 | Class. k=5 | Class. k=6 | Class. k=7 | Class. k=8 | Class. k=9 | Class. k=10 | Mean |
Lk | 103 | 102 | 500 | 103 | 2,000 | 200 | 200 | 103 | 50 | 500 | N/A |
Ck (baseline) | 106 | 103 | 102 | 103 | 103 | 102 | 102 | 1 | 10 | 102 | N/A |
Mean Acc. | 0.879 | 0.916 | 0.897 | 0.996∗ | 0.991 | 0.906 | 0.992 | 0.998 | 0.872 | 0.996∗ | 0.944 |
Dev. | 0.074 | 0.036 | 0.015∗ | 0.021 | 0.029 | 0.037 | 0.027 | 0.012 | 0.074 | 0.02 | 0.034 |
Precision | 0.852 | 1 | 0.1 | 1 | 0.942 | 0.643 | 1 | 0.985∗ | 0.291 | 1 | 0.781 |
Recall | 0.799 | 0.16 | 0.005 | 0.965 | 0.97∗ | 0.135 | 0.92 | 1 | 0.195 | 0.955 | 0.61 |
Download links
A two-part list of links to download the article, or parts of the article, in various formats.