
The Emergency Family Income (IFE) in Argentina: could it become a permanent safety net?
- Article
- Figures and data
-
Jump to
- Abstract
- 1. Introduction: the emergency family income: a one-time measure?
- 2. Dynamic microsimulation for COVID19 policy response, and projected economic scenarios
- 3. Results: cost and redistributive impact of the IFE
- 4. Conclusion: getting out of the oxymoron of a permanent emergency family income
- Footnotes
- References
- Article and author information
Abstract
Argentina adopted an early lock-down strategy to mitigate the COVID19 pandemic in March 2020. To allow households that were out of the formal labour market to abide by the lock-down, an Emergency Family Income (IFE) was given to households with no pension or formal labour income between April and September 2020. The benefit was praised for its role in reducing poverty and providing for millions of households that had lost their income, but it was discontinued after September 2020 when most of the COVID19 restrictions had been lifted. In this paper, we run counter-factual dynamic simulations of what would have been the cost and distributive impact of maintaining the IFE after September 2020, and making it a permanent benefit. We show this would have represented initially a yearly additional expenditure of nearly 1.5% of GDP, with a downward trend. This is comparable to the cost of all family benefits. We also estimate that a permanent IFE would have significantly reduced income inequality. We thus show a permanent IFE would be financially feasible, although whether it is desirable or how it should be funded is open to debate.
1. Introduction: the emergency family income: a one-time measure?
The Argentinian economy was already in a difficult situation when the COVID19 pandemic struck. There was an ongoing recession, with GDP falling -2.5% and -2.2% in 2018 and 2019, with a further recession at -1.3% of GDP for 2020 projected by late 2019 (ECLAC, 2019). Argentina had gone into a financial, currency and sovereign debt crisis in May 2018, when access to international financial markets was cut. The government signed a stand-by agreement with the IMF in June 2018. Although the agreement allegedly was of a precautionary type, the government received nearly 45 billion of US dollars between June 2018 and July 2019 from the IMF, which did not restore confidence as expected. No further disbursements were made when the then president Macri lost a primary election to the peronist party’s candidate Alberto Fernández in August 2019. In September 2019 the payment of short-term domestic debt in pesos was postponed, which meant access to the domestic financial market was also cut off. Between May 2018 and December 2019, the US dollar soared by 197% in Argentinian pesos, inflation figures reached their highest level since the 1991 hyperinflation (53.8% on average for 2019), and there was no access to financial markets or further funding from the IMF.
Despite this difficult economic situation, the Fernández administration, sworn in December 2019, adopted a strict lock-down in March 2020 to contain the spread of the COVID19 pandemic. Although it was gradually relaxed starting since late April, there were heavy restrictions on the economic activity until September. Argentina experienced therefore a depression of nearly -10% of GDP in 2020: the COVID19 pandemic thus decreased expected growth by 8.7% of GDP.1 This is in line with the impact of the pandemic in Latin America and the Caribbean, with GDP decreasing from an estimated 1.4% growth for 2020 (ECLAC, 2019), to a depression of nearly -7.7% of GDP in 2020 (ECLAC, 2021), that is, an impact of 9.1% decline in GDP.
The depression had a strong impact on Argentina’s labour market. Even though formal salaried work only experienced a small decrease due to pre-existing limitations on the possibility of laying-off workers, and to strong government transfers through the Assistance to Labour and Production program (ATP), inactivity and unemployment rates soared. Following our own computations based on the INDEC’s Continuous Permanent Household Survey (EPHc), the inactivity rate between the first and second quarters of 2020 for men aged 16 to 69 jumped from 23% to 33%; for women of those same ages, inactivity rates increased from 42% to 52% between those two quarters. Meanwhile, the proportion of independent labourers and of workers in the informal sector dropped strongly between the first and second quarters of 2020. The proportion of men aged 16 to 69 that worked in the formal sector dropped by 33% between the first and second quarters of 2020 (from 27% to only 18%). Meanwhile, the share of women aged 16 to 69 that worked in the informal sector dropped by 38% (from 21% to 13%).
The COVID19 pandemic and the non-pharmaceutical public health measures undertaken to mitigate its spread thus meant a large part of the unprotected workers of the informal sector simply lost access to labour income. Therefore, the Argentinian government implemented the Emergency Family Income (IFE) by Decree 310/2020 (24/3/2020), in order to both guarantee the effectiveness of the sanitary strategy for containing the COVID19, and limit the impact of the depression on the most vulnerable populations. Each household that had no other source of income would receive a $10,000 pesos benefit, which was equivalent to 59% of the minimum wage, or 156 USD. The two lower categories of the small independent workers scheme, the monotributo, could also earn the benefit, following Art. 2 of Decree 310/2020.
Although it originally was meant to be paid only once, and the government initially estimated it would reach 3.6 million households (Carrillo, 2020), 8.9 million households received the first payment, or 31.6% of the population aged 18 to 65 (ANSES, 2020). In the end, it became a bimonthly benefit (each household received one payment every two months), and three total payments were made: in April and May, June and July, and August and September. Following ANSES (2020), without the IFE 1.4 million people would have become poor. Also, 2.8 million people would have fallen into extreme poverty in the second quarter of 2020. This would have increased by 3 percentage points the poverty rate, and by 6 percentage points extreme poverty figures. According to the Catholic University of Argentina, in the third quarter of 2020 the IFE prevented an increase in poverty and extreme poverty rates of respectively 6.4 and 8.3 percentage points (UCA, 2020). The ATP and the IFE ended up being the two main measures taken by the Argentinian government to mitigate the economic impact of the COVID19. Together, they explained most of the 4.2% GDP increase in current transfers estimated by ECLAC (2021). The cost of the IFE alone can be estimated at around 1% of GDP, based on the number of beneficiaries informed by ANSES (2020).
The IFE was thus widely viewed as a very effective tool for limiting the increase of poverty levels during the pandemic. However, as the economy started recovering in parallel with the easing of non-pharmaceutical measures to contain the COVID19 by the third quarter of 2020, it was decided not to renew this benefit past September. As explained earlier, the Argentinian government could not get net voluntary funding neither in the domestic or international financial markets, nor from the IMF. As such, the IFE was mostly funded with money emission by the Central Bank. Even though inflation decreased significantly in 2020 (36.1%) due to the economic recession and stabilisation of the Argentinian peso, the fourth quarter of 2020 saw an up-tick in monthly inflation figures as the economy started recovering, and an increasing gap between the official and parallel exchange rates. Also, a significant point under negotiation with the IMF is primary deficit figures, pointing to the limitation of public spending.
Despite this, the Argentinian government has repeatedly stated it will reinstate the IFE if a new lock-down is needed in 2021 due to a second wave of COVID19. The IFE also prompted a debate on whether there should be a permanent universal base income, in a country where a large proportion of its population is excluded from the formal workforce. It even prompted similar measures in neighbouring countries, to the point that the government of Chile implemented in May 2020 its own Emergency Family Income, keeping the same name as its Argentinian counterpart. Other countries, such as Brazil, instituted similar government transfers at the onset of the COVID19 pandemic, but paid them for a longer time (in the case of Brazil, up until January 2021). Therefore, it is important to anticipate what would be the cost and impact of a permanent IFE, not only because it is a measure that is likely to return this austral winter if the vaccination campaign is unable to prevent a second wave of COVID19 in Argentina. But also, because it could serve as a baseline for future informality and poverty tackling policies.
The goal of this paper is to provide a counter-factual simulation of what would have been the cost and impact of keeping the Emergency Family Income as a permanent measure, assuming it either followed the evolution of the Consumers Price Index, or that of the new pension mobility formula established by Law 27609, up to 2040. It also estimates the distributive impact of the three IFE payments that were made in 2020, "now-casting" the benefit’s impact while waiting for official figures. To do this, we adapt the existing dynamic Microsimulation model for Social Security in Argentina (MISSAR) (Calcagno, 2018), to model the IFE. In Section 2 we give a brief account of the methodology used for the estimation, as well as the macroeconomic data and hypotheses we adopted in our projections. Finally, in Section 3 we show that both hypothetical alternatives for a permanent IFE have a significant but manageable cost, and would have a strong distributive impact.
2. Dynamic microsimulation for COVID19 policy response, and projected economic scenarios
2.1. Adapting the dynamic Microsimulation model for Social Security in Argentina (MISSAR) to model a universal income
As was mentioned in the introduction, the IFE was an emergency income targeted at households with no pension or formal labour income, as well as the lower categories of own-account workers. We therefore need to model not only labour-market state, in particular participation in the formal labour market, but also pension income and household composition. It is also necessary to work with heterogeneous individuals: the IFE is targeted at specific households, and was thought as a way to allow the most vulnerable households to abide by the lock-down even if they lost their labour income. Therefore, it has a strong distributive impact, so one of the outputs we are most interested in is how this benefit impacted income inequality, and what would be the distributive impact of a hypothetical permanent IFE. For that, we need to model heterogeneous individuals.
To this end, we adapted the existing Dynamic Microsimulation model for Social Security in Argentina (MISSAR), developed on LIAM2 (Bryon et al., 2015) as part of my PhD thesis (Calcagno, 2018). The objective of the MISSAR model is to simulate social security contributions as well as the most relevant benefits paid by Argentina’s National Social Security Administration (ANSES). To do so, it takes as a starting population the fourth quarter of 2014 wave of Argentina’s Permanent Household Survey (EPH). This is a randomised sample of the inhabitants of 31 Argentinian cities and metropolitan areas that represent most of Argentina’s urban population, and hence most of its inhabitants, since about 91% of Argentinians live in towns of more than 2000 inhabitants.2
From this starting representative population, the MISSAR model simulates past individual careers for each respondent to get accumulated retirement rights, and then projects this augmented dataset up to 2040, where Argentina’s National Institute of Statistics and Census (INDEC)’s 2010-2040 demographic projections end. We model plausible individual behaviour in line with individual characteristics by estimating logit behavioural models and Mincer wage equations (Mincer and Polachek, 1974) based on the EPHc 2003-2015 waves, since these track individual transitions at both a quarterly and yearly pace. The macro-level consistency of the synthetic dataset is ensured through an alignment by sorting procedure made available by LIAM2, where we assume there is a given proportion of individuals that will make a determined transition (for instance, working in the formal labour-market). We pick just enough individuals among the persons that may make this transition to reproduce these exogenous proportions, and we select in priority those who are the most likely to make this transition due to their characteristics. The end result is a synthetic quarterly panel data that spans the 2014-2040 period, from where are computed each period social security contributions and benefits depending on each individual’s situation. Calcagno (2018) describes extensively the methodology of the MISSAR model, and gives a token of its results with social security projections using May 2018 legislation.
The MISSAR model is thus an adequate tool for modelling the IFE. The synthetic dataset it creates includes individual transitions in the labour market, labour income, socio-demographic transitions (birth, death, creation and dissolution of couples, marriage, divorce and education levels), social security benefits, and household composition. All this information is available at the individual level with heterogeneous individuals, while the modelled dataset is at the same time representative of the Argentinian population as a whole. It is then possible to combine an aggregate-level study of a permanent IFE’s future cost and coverage, with an individual-level estimation of the benefit’s distributive impact. The macro-level consistency of our IFE simulations is attained by calibrating our total modelled IFE benefits between April and September to the actual cost of these benefits deduced from ANSES (2020). Its individual level simulation estimates the impact of the IFE on income distribution.3
2.2. Methodological limits
Adapting the existing MISSAR model to the simulation of the IFE has however its caveats. The most significant one is that the IFE is a household-level benefit: only one is given per dwelling, and none of the household members can have a pension or a formal source of income (except for small own-account workers in the two lowest categories of the monotributo regime). A short-coming of the MISSAR model, designed primarily for computing retirement benefits that are mostly independent from household composition, is that it imperfectly simulates households. The initial version of the MISSAR model described in Calcagno (2018) had nearly 18% of simulated households with no labour or pension revenue, while only 3% of households surveyed in the fourth quarter of 2014 EPHc were in this situation. There has been a methodological change in the EPH starting in 2016, where in particular they stopped inputting missing values of labour income for working individuals (INDEC, 2017), so it is tricky to know for sure what is the actual proportion of households with no labour or pension income. It is possible that the actual figure of households with no labour or pension income is higher than 3%, but it certainly does not reach 18% of households as originally modelled in Calcagno (2018).
Still, we adopted a series of methodological adjustments that brought down to 8% the share of simulated households with no labour or pension income in 2015. First, we toughened the pre-requisites for a young adult to leave the parental household: only those older than 24 and working are compelled to leave the household by the model. We then allowed for young adults to go back to their parent’s household if they are single and not working, up to age 40. Finally, we tweaked our labour-market module so that informal workers are selected in priority among households that have no member that receives a retirement benefit or a formal source of income. We thus lower the proportion of households where all adults are either unemployed or inactive, something that cannot be attained even through our estimated logit behavioural equations. These behaviours are also logical, as they factor in family assistance and the fact each household needs at least one source of revenue in the short term.
The end-result is Figure 1. First, in Figure 1a, we see an increase in households with no labour or pension revenue between 2015 and 2025, as a consequence of a more difficult access to retirement starting 2017 and the onset of the ongoing economic crisis, that started in 2018; this results in 15% of households simulated with no labour or pension income in 2026. The proportion reaches 0% for the months where the IFE was paid. A projected increase in labour-market participation, as detailed in our scenarios in Section 2.3, along with the adjustment measures included in the MISSAR model allow for a decrease in households with no labour or pension revenue, which reach 2% of the total in 2040 in our central economic scenario. This results in a simulated access to the IFE of 36% the second quarter of 2020, which is slightly higher than the 31.6% of households that got the IFE following ANSES (2020), as shown in Figure 1b. This means the high share of households modelled with no labour or pension income as shown in Figure 1a leads to an overestimation of the IFE’s reach.
This being said, there are other methodological limits to modelling the IFE benefit with the MISSAR model. As pointed out in Calcagno (2018), "it models individual behaviour following a deterministic setting, where it is the characteristics of the individual that make him or her more likely to experience a given transition. [In this setting,] there is no maximisation strategy behind observed behaviour. (…) Our model thus cannot have endogenous labour supply, income, retirement decisions that would change the aggregate proportions of, say, formal workers based on individual maximisation programs. The simulated behaviour is thus not robust to the Lucas critique, in that we cannot endogenously model the reaction of individuals to a change in legislation." (Calcagno, 2018, p. 504).
A permanent IFE could be an incentive to avoid working in the formal labour market, so it raises the question on how to accomodate this probable change in individual behaviour with the deterministic setting adopted in the model. In the case of the Universal Child Benefit (AUH), implemented in 2009 for children with parents that are out of the registered labour force, Garganta and Gasparini (2015) found this benefit may have discouraged workers from the lower income deciles with children to work in the formal sector. Doing so would have meant earning contributive child benefits, which are lower than the AUH, and for those workers of the lower income deciles the AUH represented a substantial share of their revenue. A similar behaviour is likely in case of a permanent IFE, although it could be included in the model by assuming higher levels of informal labour than in a scenario without a permanent IFE.
Another important limit is that the starting dataset, the EPH household survey, does not include information on whether an independent worker is formal or not. As explained in Calcagno (2018), we pick among independent workers those most likely to be formal and, among these, those that are likely among the lower categories of formal independent labour. Since labour income is moreover under-reported in the EPH (Calabria and Gaiada, 2019), we are probably overestimating the proportion of formal own-account workers that are eligible to the IFE, as per Art. 2 of Decree 310/2020. Calabria and Gaiada (2019) even point out own-account workers in Argentina typically under-declare their income by a more significant margin than wage-earners, as they may fear declaring their actual revenue in a survey may bring them trouble later on with tax authorities.
Finally, this under-reporting of labour and pension income in the EPH may under-estimate income inequality, as typically higher-earning individuals are the ones who under-report their income the most; and over-estimate the impact of the IFE, as total disposable income in the EPH is lower than in reality. This methodological limitation is problematic when measuring poverty levels, and as such some authors correct it with alternative sources of data, mostly coming from National Accounts (Calabria and Gaiada, 2019), following the seminal papers of Altimir (1979) and Altimir (1987).
However, a large proportion of the literature prefers to measure income inequality in Latin America with raw data. This is the case of CEDLAS and The World Bank (2014) and UN’s Economic Commission for Latin America and the Caribbean (ECLAC). As pointed out by Atuesta Montes et al. (2018), p. 65, “this procedure is subject to a series of limitations that led the ECLAC to stop using it for estimating regionally comparable poverty and income inequality series. Some of the limitations include the precariousness of data taken from Income and Expenditure Accounts in some countries of the region, the weakness of the “unit elasticity” hypothesis, and the sensitivity of results to the characteristics of data (Villatoro, 2015).” The “unit elasticity” hypothesis in particular means assuming that “under-reporting depends more on the type of income, and not on its level, and that it follows a unit elasticity framework across income levels, except for property income” (Villatoro, 2015, p. 10).
Since we study in this paper the distributive impact of the IFE, we decided to follow this literature and not correct under-reported income in the EPH. This correction would however be important for studying the poverty alleviation impact of a permanent IFE, and is thus left for future work.
Despite these limits, which could partially explain why we simulate a proportion of households that get the IFE that is higher than what is reported by ANSES (2020), we can see in Figure 1b that the model still reproduces an IFE coverage that is close to measured values. Although some of these methodological limits can be reduced with further work, simulated IFE coverage is realistic enough to evaluate what would be the cost and impact of a permanent universal income in Argentina. Before carrying out this analysis in Section 3, we will lay out in Section 2.3 the macroeconomic situation and scenarios that is used for the macro-level calibration of the MISSAR model.
2.3. Economic scenarios
In Figure 2, we show the evolution of labour-market participation in Argentina of men (Figure 2a) and women (Figure 2b) ages 16 to 69. We use historical values drawn from the EPH between September 1974 and the third quarter of 2020, and then assume three distinct scenarios that range from the fourth quarter of 2020 to 2040. The five labour-market states laid out were thought with the simulation of retirement rights in mind in the MISSAR model. Formal wage-earners are those that report in the EPH that they contribute to a retirement scheme. Independent workers of the formal sector are those that own medium or large companies, or that have a high educationor skill level in their occupation, following the methodology by Maurizio (2012). The rest of independent workers, as well as wage-earners that do not contribute to a pension system, are part of the informal sector. The unemployed category is directly measured by the EPH following the ILO definition. The remainder of the population is considered inactive.
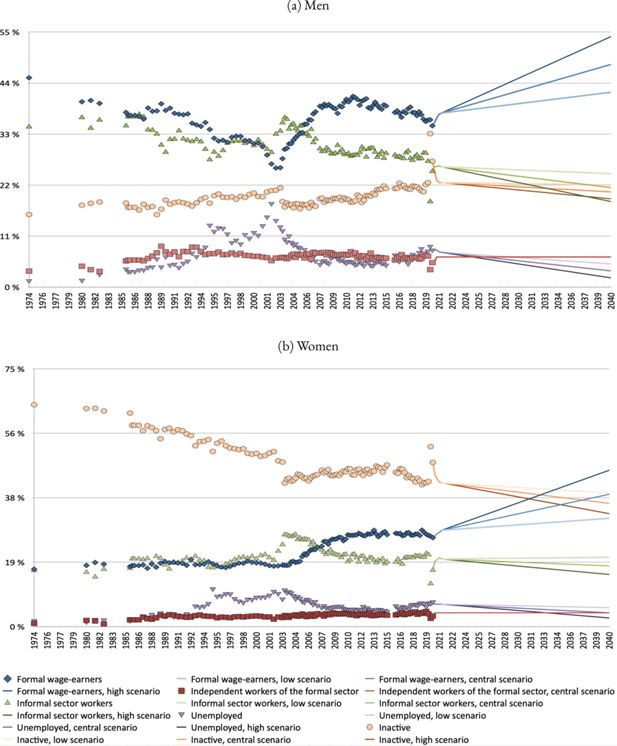
Labour-market participation rates for people aged 16 to 69, past rates, and projected scenarios (1974-2040). Source: historical values taken from EPH survey (1974-2020).
We can see that the proportion of male formal wage-earners remained around 38% between 2008 and 2018, and that a downward trend started in the second half of 2018 with the beginning of the crisis, reaching 35% in the third quarter of 2020. The fall in formal employment implied higher levels of unemployment and inactivity, since informal labour also decreased during the crisis. The share of female registered wage-earners has remained stable at around 27% of the population since 2011. Up until the COVID19 pandemic, there was an increase in female labour force participation exacerbated with the 2018 crisis. The fall in inactivity rates meant more women became active, but as informal workers or part of the unemployed.
Figure 2 also shows the brutal impact of the COVID19 pandemic in the labour market. The second quarter of 2020 saw inactivity rates soar for both men and women, at the expense primarily of informal labour, although independent labour in the formal sector also declined. We can see the COVID19 shock was partially absorbed by the third quarter of 2020, with inactivity rates dropping rapidly, although men returned to activity faster than women. The situation had not however reached its pre-pandemic levels, which explains why the IFE was maintained up until September 2020.
We finally assume the labour-market situation should recover in two steps. First, that the COVID19 shock should be fully absorbed throughout 2021. Second, that the proportion of formal wage-earners should increase for both men and women, at the expense of unemployment rates and informal labour. In the case of women, we expect a more important increase in their participation in the formal labour-market as part of their increasing activity rates. Still, we propose labour-market scenarios that are distinctly different from one another, so that our analysis could cover a broad array of possibilities. In any case, the model can be run with other macroeconomic scenarios for the 2020-2040 period. We nevertheless leave this to future work.
In accordance with our expected recovery of the labour market, we assume wages will recover from their fall since the onset of the 2018 crisis. We saw in Figure 2 that this recession only moderately hit formal labour, and that the COVID19 shock was mostly absorbed through a temporary fall in informal labour. The 2018 crisis however, with its surge in inflation figures, caused a fall in labour income of 16% between the first quarter of 2018 and the fourth quarter of 2020. The historical data we took from Calcagno (2018) shows this is the worst fall in formal income since the 2001 crisis (-29% between the fourth quarter of 2001 and the first quarter of 2003), and that formal wages are today at levels akin to the late 1990s or 2006. It is thus logical to assume real wages will recover, and we suppose different paces in Figure 3. We go from a very conservative pessimistic scenario where formal wages do not recover their pre-crisis levels by 2040, to an optimistic scenario where wages reach these by 2025.
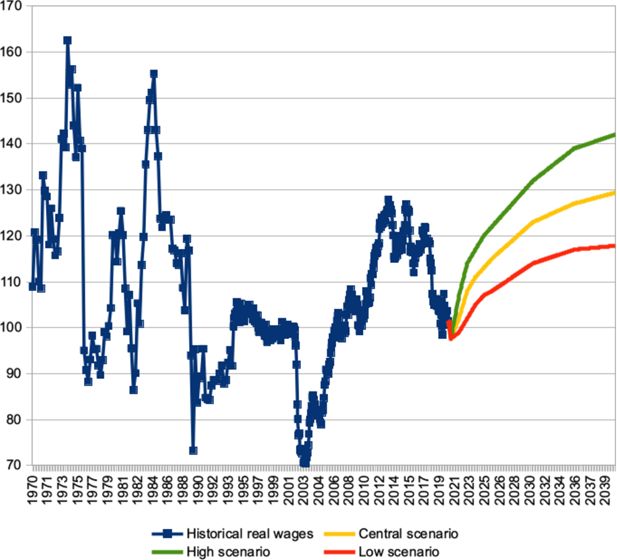
Quarterly real wage evolution, by scenario (1970-2040). Sources: for historical values, see Calcagno (2018). The base period is December 2006.
Moreover, we follow the French Pensions Advisory Council (COR) methodology for GDP growth projections. We assume GDP growth in the short term, with a varying share of labour compensation in the GDP (COR, 2018); and in the middle to long run, we assume a fixed labour compensation share of GDP. We thus make growth follow labour productivity gains, which we assume are reflected on wages, and working population growth (COR, 2016). This results in varying levels of economic recovery in 2021 (7%, 9% and 11% depending on the scenario) followed by varying long-term levels of GDP growth (1.5%, 2.3% and 2.9% of average real GDP growth). The resulting optimistic, central and pessimistic macroeconomic scenarios are depicted in Figure 4.
Finally, the MISSAR model estimates future social security benefits. To this end, we compute pension indexation as determined by the current Law 27609, depending on the adopted macroeconomic scenarios. This pension mobility formula, in effect since March 2021, makes benefits follow nominal wage evolution by 50%, and the other 50% depends on the variation of ANSES fiscal income divided by total social security benefits. We assume ANSES fiscal income represents a little over 3% of GDP, and estimate with our model the evolution of total retirement benefits. With this, we get in Figure 5 a projection of real pension mobility by economic scenario. This is not only important for the simulation of social security benefits in the MISSAR model; it is directly used when estimating a permanent IFE benefit, as one of the hypotheses that we will develop in Section 3 is that such a hypothetical benefit would be indexed following the pension mobility Law 27609.
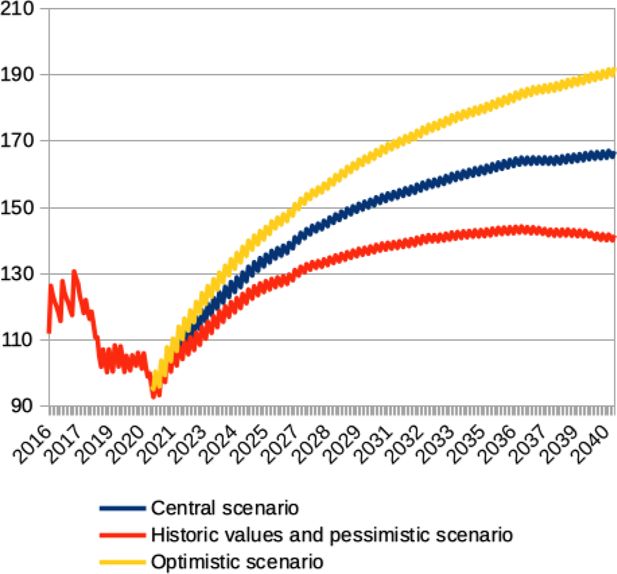
Real pension mobility by economic scenario (2016-2040). Note: The base period is December 2020.
We can see that the change in March 2018 of the pension mobility Law, together with the onset of the economic crisis that same year and, to a lesser extent, the increase of benefits by Decree throughout 2020, lead to a drop in pensions, with on average a decrease of nearly 19% between December 2017 and December 2020.4 We estimate that the expected economic recovery, together with the new pension mobility Law 27609 that effectively indexes social security benefits on both growth and wages, will regain the purchasing power of these benefits in all economic scenarios, although with varying speeds. December 2017 levels should be recovered by 2023, 2024, and 2025 in our optimistic, central and pessimistic scenarios. Between 2025 and 2040, pensions should further increase in real terms by respectively 33%, 23% and 11% in these scenarios. This will have an impact on Argentina’s social security system, although this point will be further studied in a future paper.
3. Results: cost and redistributive impact of the IFE
3.1. A permanent IFE would have a significant, but declining, cost
We have shown in Figure 1 that we simulate a coverage level of a permanent IFE that is akin to what was reported by ANSES (2020). We also explained that our aggregate simulations of the cost of the IFE are aligned to an approximation of the measure’s cost from April through September 2020 following ANSES (2020). We assume that, if the IFE had been also paid the remainder of 2020, it would have maintained its original value of $10,000 pesos; but we then suppose that starting from the first quarter of 2021, its value would have been indexed either following the Consumer Price Index, or the pension mobility Law 27609, whose real evolutions are depicted in Figure 5. The resulting estimated cost of the IFE is depicted in Figure 6.
First of all, we find that, had the IFE been maintained throughout the fourth quarter of 2020, its total cost would have reached 1.4% of GDP. This means paying the IFE three more months would have cost an additional 0.4% of GDP. Since we estimate based on data from ANSES (2020) that the IFE cost roughly 1% of GDP in 2020, our estimated cost of paying the IFE up until December 2020 is logical: it would have been paid for 9 months instead of 6.
From this point, the total cost of the IFE is closely related to the indexation method that is adopted. In the scenario where it follows inflation, we also assume it is gradually incremented between the fourth quarter of 2020 and the third quarter of 2021 so that, in that quarter, it reaches the same real value it had in the third quarter of 2020. With this hypothesis, the benefit would represent roughly 1.7% of GDP in 2021. It then would stay frozen in real terms, which by 2030 would decrease the share of the IFE to 0.5%, 0.6% and 0.8% of GDP in respectively our optimistic, central and pessimistic scenarios. As seen in Figure 1b, after that date IFE coverage drops significantly, as a consequence of the expected increase of the formal labour force depicted in Figure 2 which further reduces the cost of this hypothetical permanent IFE to 0.1% or 0.2% of GDP depending on the economic scenario.
Meanwhile, if we index this hypothetical permanent IFE with the pension mobility formula, the evolution of its cost is smoother. It reaches 1.5% of GDP in 2021, it goes to between 1.6% and 1.4% of GDP in the optimistic and pessimistic economic scenarios, then gradually decreases its GDP share starting 2025. As shown in Figure 5, the real increase in pensions is significantly higher in the optimistic economic scenario as compared to the pessimistic projections. This is why the estimated share of the IFE as a percent of GDP is usually higher in our optimistic economic scenario, despite having a lower coverage. By 2030 it goes down to 1.1% of GDP, and as the coverage of the IFE further drops, it goes down to 0.2% of GDP by 2040. Also, after 2023, its cost is higher than adopting an inflation indexation in all our economic scenarios. This means that, apart from the initial raise to make the IFE match its third quarter of 2020 values, the Law 27609 pension indexation increases benefits consistently over inflation figures in our economic scenarios, as shown in Figure 5.
These results show the cost of a hypothetical permanent IFE follows a similar dynamic in both indexation hypotheses. Even if we take the more generous on average pension mobility indexation, the cost of a permanent hypothetical IFE would not go beyond 1.6% of GDP, and would gradually decrease as the economy recovers. This is comparable to the cost of all family benefits paid by ANSES, which represented 1.5% of GDP in 2019. A permanent IFE would thus represent a significant but not exorbitant budgetary cost, which would additionally decrease over time. For instance, the tax on checking account payments (or "Check" tax), which should have been eliminated following the 2017 fiscal reform Law 27430, represents 1.6% of GDP. Also, the one-time extraordinary contribution of the 12 thousand wealthiest Argentinian tax-payers to fund measures taken to face the COVID19 pandemic is expected to represent nearly 1% of GDP.
Our results show that a permanent IFE could be funded if there was the political will and social consensus to obtain supplementary public revenues to finance this kind of progressive benefit. Indeed, the cost of a permanent IFE is too high for it to be implemented without at the same time increasing public income by an equivalent amount, be it through new taxes, lower tax exemptions, or by achieving lower levels of tax evasion. Even if a permanent IFE would at least partially repay itself by enhancing growth, it would still need to be funded in the short term. We end this paper by estimating what would be the distributive impact of implementing a permanent universal base benefit such as the IFE in Argentina.
3.2. A permanent IFE would strongly decrease inequality
In Figure 7, we compare income inequality with and without a permanent IFE benefit. Households with neither labour nor IFE income are excluded from this analysis. This excludes households with only pension income; capital income is not included in the model. We measure the Gini index computed on labour income with and without the IFE. We also compute the share of labour income, with and without the IFE, that is received by the 10% wealthiest and poorest households. The determination of the top and bottom 10% households as ranked by their income is done with respect to total income (labour, pension and family benefits). Household income is equivalised following CEDLAS and The World Bank (2014), who adopted the calibration established by Deaton and Zaidi (2002).
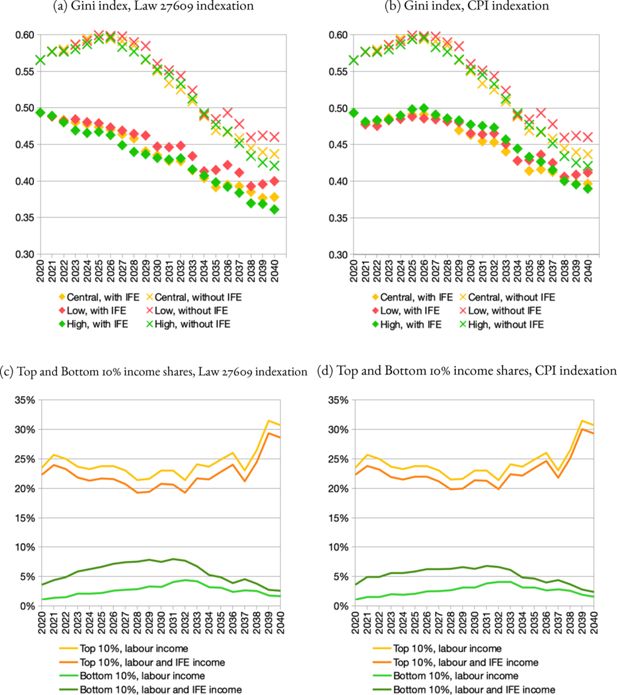
Gini index and top and bottom 10% share of income with and without a permanent IFE benefit (2020-2040). Note: Households with neither labour nor IFE income are excluded from this analysis. For the share of the top and bottom 10% in thetotal of a given income type however, the determination of the wealthiest and poorest households is always done with respect tototal income (labour, pension, IFE and family benefits). The Top and Bottom 10% shares are only computed in the centraleconomic scenario. Household income is equivalised following CEDLAS and The World Bank (2014), who adopted thecalibration established by Deaton and Zaidi (2002).
It is necessary to remind here that, as was explained in Section 2.2 and underlined in Figure 1a, the MISSAR model tends to overestimate the proportion of households with no labour or pension income. As such, the simulated Gini index levels are likely higher than their actual values, because the Gini index is very sensitive to null values, and we need to factor in households with no labour income if we want to study the distributive impact of the IFE. The values estimated for 2020 (0.49 and 0.56 with and without the IFE) are significantly higher than the Gini index we computed on labour income for the fourth quarter of 2014 EPH wave (0.43), using the methodology of CEDLAS and The World Bank (2014). This bias is however less important when computing the top and bottom 10% income shares, as we computed on the fourth quarter of 2014 that these deciles captured respectively 22% and 2% of total labour income (Calcagno, 2018).
Despite these limits, we can see in Figure 7 that the distributive impact of the IFE was large in 2020, and would have continued to be so had it been made permanent. Without the IFE, the Gini index would have been 6 percentage points higher in 2020, and would have started decreasing significantly from 2030 onward, when we simulate a lower proportion of households with no income. With a permanent IFE, we would have a steady reduction of income inequality if we follow the Law 27609 indexation as shown in Figure 7a. In our central scenario, the Gini index would have gone from 0.5 in 2020 to 0.37 in 2040. Without a permanent IFE, the Gini would also decrease from 0.56 to 0.44. With the IFE indexed on inflation, the Gini reduction would be smaller as depicted by Figure 7b (reaching 0.4 in 2040). Further refinement in household modelling is needed to get better simulated Gini indexes; still, these results give a token of the distributive impact the IFE has had in 2020, as shown by its capacity of mitigating the increase in poverty levels (UCA, 2020; ANSES, 2020); and the high distributive impact a similar benefit could have in the future.
The distributive impact of the IFE is however best measured by how it affects the income share of the top and bottom 10% deciles. Without the IFE, simulated labour income captured by the top 10% fluctuates around 25%. With a permanent IFE benefit, this share drops by 2.1 percentage points on average, when it is adjusted according to the pension mobility Law 27609, as depicted by Figure 7c. When the IFE benefit is frozen in real terms, the distributive impact of the IFE decreases over time, as shown by Figure 7d. Adjusting this permanent IFE following inflation figures would decrease the top 10% income share by 1.9 percentage points in 2021, but only 1.4 points in 2040. The average decrease between those years would be of 1.6 percentage points. A permanent IFE would thus decrease the income share of the top decile.
The strongest impact is however apparent in the income captured by the bottom 10%. We model they only get 1.4% of labour income in 2021, when they used to get 2.6% of labour income the fourth quarter of 2014. This share increases after 2030 as we model an increase in formal and informal labour, as well as a lower proportion of households modelled with no income, reaching 4% in 2040. With a hypothetical permanent IFE, this share increases to 4.4% in 2021, and increases slightly over 7% in following years, as shown by Figure 7c. The distributive impact is higher when the IFE follows the pension mobility Law 27609, although in this case the difference is less significant than with the other income inequality metrics shown in Figure 7. Finally, the IFE impact in the bottom 10% decreases over time, although it is mostly explained by a shift in its distribution in income decile as will be shown in Figure 8a.
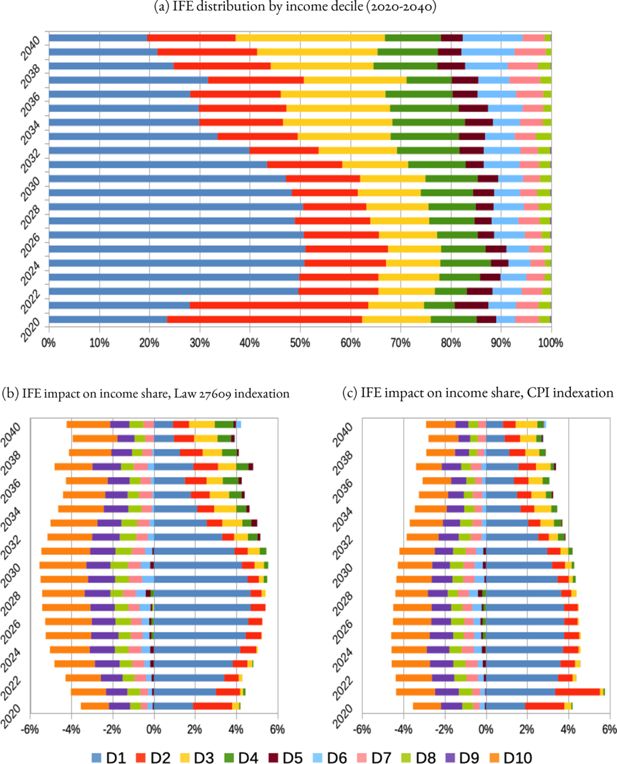
Distributive impact of a permanent IFE by income decile (2020-2040). Note: Households with neither labour nor IFE income are excluded from this analysis. Income deciles are however always computed with respect to total income (labour, pension, IFE, and family benefits). The income deciles are only computed in the centraleconomic scenario. Household income is equivalised following CEDLAS and The World Bank (2014), who adopted thecalibration established by Deaton and Zaidi (2002). Reading example for Figure 8c in 2021, a permanent IFE indexed on pensionmobility Law 27690 would increase the first decile income share by 3.4 percentage points, when compared to its labour incomeparticipation.
Finally, we also study how the IFE is distributed among income deciles, and how it affects their participation in total (labour + IFE) income in Figure 8. Figure 8a shows a permanent IFE would be mostly received by the poorest households, although the participation of higher income deciles would gradually increase over time. There is most likely an effect of size: large households may be ineligible because one of their members earns a retirement pension or a formal wage, but fall in the lowest deciles due to the high number of dwellers. Meanwhile, smaller households may cumulate informal labour income, or income from smaller monotributo categories, and the IFE. The fact the share of the IFE earned by higher income deciles increases over time in our simulations is most likely an artefact linked to the gradual fall in simulated households with no labour or pension income as shown in Figure 1a.
Despite this limitation, we can still see most of the simulated IFE benefits are given to the poorest households. Between 2020 and 2030, 89% of permanent IFE benefits would be given to the 50% poorest households; and 64% to the bottom 20% households. Even though we simulate a shift of IFE coverage to higher income deciles, even by 2040 we estimate 82% of the benefits would be received by the bottom half of the income distribution, and 43% by the two lower deciles. This shows the IFE is mostly a targeted benefit, but not restricted to the poorest households.
Figure 8b and cshow the difference between each decile’s share of labour and IFE benefits, and the proportion of labour income earned by it. They both show the IFE has a strong positive impact in the income share of the bottom 20% income deciles, while also reducing the top 20% income deciles shares. Between 2020 and 2030, with a permanent IFE indexed following pension mobility, the labour and IFE income share of the poorer two income deciles is 4.8 percent points higher than their labour income share; while the labour and IFE income share of the top 20% decreases by 3.2 percent points when the IFE is incorporated. We can also see the IFE is mostly neutral for the middle of the income distribution (deciles 4 to 6).
When comparing the distributive impact of the permanent IFE variants, defined by their indexation formula, we can see in Figure 8c the impact is stronger in 2021 if this permanent IFE is discounted following inflation, but is then lower than discounting with the Law 27609 pension mobility formula (Figure 8b) in the following years. This is in accordance with Figure 6, which shows an IFE indexed in the CPI would be more expensive than an alternative indexed on pension mobility in 2021, but that it would be cheaper than indexation on pension mobility from 2022 onward.
This analysis by income decile shows that a permanent IFE would have a significant distributional impact past the top and bottom income deciles, while also being mostly neutral for the middle of the income distribution. This shows a permanent IFE would improve the livelihood of poor households, and would not be restricted to those in extreme poverty.
All these elements show, despite the methodological limits laid out in Section 2.2, that the IFE had a strong distributive impact in 2020, and a similar long-term policy would also significantly better the livelihood of the most vulnerable households, and decrease income inequality as a whole.
4. Conclusion: getting out of the oxymoron of a permanent emergency family income
As a conclusion, this paper is at the intersection of various research questions and political debates. The Emergency Family Income was thought as an integral part of the non-pharmaceutical government interventions taken to contain the COVID19 pandemic in March 2020. Without the IFE, it would have been impossible both pragmatically and morally to ask Argentinians with no formal income to stay home. The non-renewal of this benefit past September 2020 also followed this same logic: since by October most restrictions to economic activities had been lifted in the country, there was no further need to ask the population to stay home, so the IFE could be discontinued. Finally, the official promise to reintroduce the IFE in case of a second lock-down underlines that the current political consensus is that the IFE should function as a tool to fight the COVID19 pandemic, not as a permanent measure.
There is no doubt the Emergency Family Income had a strong distributive impact and helped quell poverty and extreme poverty during the worst recession the country experienced since the 2001-2002 crisis. We show in this paper that keeping the IFE and making it permanent would have had a significant cost, slightly above 1.5% of GDP, similar to all yearly family benefits. We however also show this cost would decline in time, assuming there is economic growth and lower levels of unemployment and inactivity rates. This also means that, if there is a second lock-down in Argentina this austral winter, then such a measure would cost roughly 0.4% of GDP per quarter, which is not an insurmountable amount. Also, despite the methodological limits we faced when adapting the Dynamic Microsimulation Model for Social Security in Argentina (MISSAR) to the modelling of the IFE, we show it would have a positive, lasting, and significant distributive impact.
There are certainly methodological limits in this analysis. Most importantly, further work should include a better modelling of household composition, so as to get closer to the actual proportion of dwellings with no labour or pension income as measured in the EPH survey. This would not only allow for a better modelling of a permanent IFE’s future cost and distributive impact. It would also make it possible to start modelling poverty and extreme poverty in the MISSAR model, and gauge the impact of a permanent IFE on these statistics.
Another limit of the MISSAR model is that both aggregate labour supply and GDP growth is exogenous: we do not make these depend on the existence and amount of social security benefits. The IFE was designed to allow people not participating in the labour force in the midst of the pandemic; however, making it permanent could lower formal labour supply, since the benefit is not compatible with most forms of formal labour. On the other hand, it would strengthen our analysis to lift the hypothesis that GDP growth is independent from social security benefits: the contribution to consumption, as well as the reduction of poverty levels and of inequality as a result of a permanent IFE, would surely increase long-term GDP growth, and may partially offset its financial cost. The MISSAR model would thus gain from having its macroeconomic hypotheses estimated by a distinct macro model where, among other elements, a hypothetical permanent IFE could have an impact on projected growth.
Finally, we have studied the impact of the IFE on income inequality without studying its funding. In the midst of the emergency caused by 2020 COVID19 pandemic, with virtually no access to international or domestic financial markets and no time to levy new taxes, the IFE was effectively funded through money emission. The consequence of this emission on inflation may not be trivial. If not through excessive demand, excessive money emission could impact on the exchange rate. In this case, the benefits of the IFE could have been partially offset by an increase in inflation.
Given present and projected fiscal conditions, it is unrealistic to assume that a permanent IFE could be funded only by money emission, debt, or with funds taken from the general budget of the State, with no increase in government income. To get out of the oxymoron of a permanent emergency family income, the funding of such a measure is fundamental. If such a measure is funded through regressive measures such as an increase in the VAT, it loses most of its significance. Advocates of a permanent Universal Base Income such as Fernández Pastor (2020) know this, and propose raising a range of progressive taxes such as increasing employers contributions or reintroducing a inheritance tax, and unifying this base income with other existing benefits. Since the one-time solidarity contribution of Argentina’s biggest fortunes should represent roughly 1% of GDP in 2021, it is clear the wealthiest Argentinians have the ability to pay for such a benefit.
All in all, we have shown extending the IFE in time, although certainly costly, would have been financially feasible and would have carried out a strong distributive impact. During the six months it was in place, it was very effective in reducing poverty, and most certainly helped in implementing an initially strict lock-down and containing the COVID19 pandemic. The current consensus is that the IFE is part of the non-pharmaceutical government interventions to fight COVID19, and it currently does not have the vocation of becoming a permanent social security measure. We believe this consensus is not self-evident. The questions going forward are whether it is desirable to spend 1.5% of GDP the first years in an extended IFE; if so, who should pay for this expense; whether there are other priorities for government spending; if it is even desirable to give an unconditional income to households outside of the formal labour force; and if this kind of benefit would be the best way to contain and, eventually, eradicate extreme poverty. The aim of this paper is to contribute to this debate, which we believe the Argentinian society needs to have.
Footnotes
1.
Source: estimation based on the Monthly Estimator of Economic Activity (EMAE) from the National Institute of Statistics and Census (INDEC).
2.
Source: own calculus based on 2010 Argentinian Census data (Censo Nacional de Población, Hogares y Viviendas, 2010), INDEC.
3.
We use the Zenodo digital repository service for long-term preservation of our code. The DOI link is: https://doi.org/10.5281/zenodo.4665857. The corresponding GitHub release can be found in the following link: https://github.com/leonardo-calcagno/MISSAR/releases/tag/v1.31.
4.
On March 2020, pension increases were partially granted through a fixed-sum benefit. As such, real pension mobility was higher for lower benefits: we thus estimated the average nominal increase on March 2020 was of 8%, for the sake of this Figure. This approximation is not taken into account in the MISSAR model, as it simulates pension mobility with the lump-sum in March 2020.
References
- 1
-
2
Income distribution statistics in Latin America and their reliabilityReview of Income and Wealth 33:111–155.https://doi.org/10.1111/j.1475-4991.1987.tb00667.x
-
3
Boletín IFE I-2020: Caracterización de la población beneficiaria. Tech. rept. Dirección General de Planeamiento, Argentina Presidencia, Argentinahttp://observatorio.anses.gob.ar/noticia/boletin-ife-i-caracterizacion-de-la-poblacion-beneficiaria-348.
-
4
Herramientas para el análisis de las desigualdades y del efecto redistributivo de las políticas públicasDocumentos de Proyectos, CEPAL 1.
-
5
Liam2 user guide. Tech rept. Release 0.11.0. Federal Planning Bureau. Economic analyses and forecasts
-
6
Análisis del Sistema Integrado Previsional Argentino (SIPA) a diez años de su creación: cobertura, distribución y tasa de sustitución [ponencia]LIV Reunión Anual de La Asociación Argentina de Economía Política, Bahía Blanca, Argentina 1.
- 7
- 8
-
9
A guide to SEDLAC, socio-economic database for Latin America and the Caribbean. Tech. rept. Centro de Estudios Distributivos, Laborales y Sociales, Universidad Nacional de La Plata; The World Bank’s LAC poverty group (LCSPP)http://www.cedlas.econo.unlp.edu.ar/wp/en/estadisticas/sedlac/metodologia-sedlac/#1496251194788-b13ca6a6-a791.
-
10
Scénarios économiques pour les projections du Conseil d’orientation des retraites: méthodede construction et enjeux. In: Conseil d’Orientation des Retraites (ed), Séance plénière du 27 janvier 2016 9 h 30. Direction générale du Trésor
-
11
Rapport annuel du COR. Tech. rept. Conseil d’Orientation des Retraites
-
12
Guidelines for constructing consumption aggregates for welfare analysisWorld Bank Publications 135 :1.
-
13
Actualización de proyecciones de crecimiento de América Latina y el Caribe en 2019 y 2020. Tech. rept. Economic Commission for Latin America and the Caribbean, Santiagohttps://www.cepal.org/es/comunicados/persiste-la-desaceleracion-generalizada-america-latina-caribe-2019-se-espera-un.
-
14
Balance Preliminar de las Economías de América Latina y el Caribe, 2020. Santiago: Economic Commission for Latin America and the Caribbean
- 15
-
16
The impact of a social program on labor informality: The case of AUH in ArgentinaJournal of Development Economics 115:99–110.https://doi.org/10.1016/j.jdeveco.2015.02.004
-
17
No respuesta de ingresos en la Encuesta Permanente de Hogares. Tech. rept. Instituto Nacional de Estadísticas y Censos, Buenos Aireshttps://www.indec.gob.ar/ftp/cuadros/sociedad/nota_EPH_ingresos_06_17.pdf.
-
18
Labour Informality in Latin America: The case of Argentina, Chile, Brazil and PeruSSRN Electronic Journal 46 :1.https://doi.org/10.2139/ssrn.2062337
-
19
Family investments in human capital: Earnings of womenJournal of Political Economy 82:S76–S108.https://doi.org/10.1086/260293
-
20
Observatorio de la Deuda Social Argentina, Universidad Católica ArgentinaInforme de avance: Deudas sociales en la argentina 2010-2020. crisis del empleo, pobrezas y desigualdades en el contexto covid-19, Observatorio de la Deuda Social Argentina, Universidad Católica Argentina, 12.
-
21
Ajuste de Los ingresos de las encuestas a LAS cuentas nacionales: Una revisión de la literaturaSerie Estudios Estadísticos, CEPAL 91 :1.
Article and author information
Author details
Funding
No specific funding for this article is reported.
Acknowledgements
Not applicable.
Publication history
- Version of Record published: August 31, 2021 (version 1)
Copyright
© 2021, Calcagno
This article is distributed under the terms of the Creative Commons Attribution License, which permits unrestricted use and redistribution provided that the original author and source are credited.