
Economic and Distributional Impacts of Covid-19 Economic Shocks on Women in Senegal
Abstract
Like other African countries, Senegal has been hit by Covid-19 and has implemented measures to contain the epidemic. These measures impact men and women differently, mainly via the impacts on the labour market. We simulate the economic shocks in a computable general equilibrium (CGE) model to assess the economic impacts. We capture the gendered impact on women’s employment by linking the CGE model with a micro-simulation employment module. Furthermore, we assess the impact on poverty and inequality by executing a distributive analysis with a sequential top-down layered micro-simulation households module. The results show that the Senegalese economy suffers from Covid-19 measures with a decrease in gross domestic product by 5% and 7% in the moderate and severe scenarios, respectively. While most sectors are negatively affected, some benefit from the increase in foreign demand (e.g., for certain agricultural products). In terms of employment, unskilled workers are the most affected group. Female workers are relatively less affected than male workers due to the predominant presence of women in the agricultural sectors. Indeed, the increased foreign demand for agricultural products positively affects the agricultural sectors. However, poverty increases at the national level for all households, especially for rural households and households living in urban areas except Dakar. Specifically, the poverty gap and severity for rural households increase more than for urban households.
1. Introduction
The coronavirus pandemic (Covid-19) had devastating global health and economic impacts in 2020, and its effects are likely to be felt well into the future. It has had and will continue to significantly impact the health and well-being of many vulnerable groups (OECD, 2020b) and household living standards (Egger et al., 2021). Women are particularly affected by crises. Indeed, the impacts of crises are never gender-neutral, and Covid-19 is no exception. Women tend to earn less than men and work in less skilled jobs. Furthermore, they are burdened with unpaid care and domestic work, which forces them to withdraw from the labour market (Azcona et al., 2020; OECD, 2020a).
With containment measures to combat Covid-19, at the beginning of May 2020, 72% of domestic workers had lost their jobs, of which 80% are women. Additionally, women are strongly represented in the sectors directly affected by the measures to limit the spread of the virus, such as the hotel and catering sector and the domestic work sector (Azcona et al., 2020; World Trade Organization (WTO), 2020). Due to their pivotal role in the family, women, to a greater extent than men, are the main victims of the measures taken to prevent the spread of the disease. It is mainly women who care for the children when the schools are closed, significantly increasing their domestic workloads (Adams-Prassl et al., 2020; Burki, 2020; OECD, 2020a; West Africa Network for Peace Building (WANEP), 2020).
Past experiences of other pandemics, such as Ebola, have shown that women suffer more than men. For instance, during the Ebola crisis of 2014, restrictions on mobility severely affected the livelihoods of female traders in West Africa. According to Burki (2020), they were hit harder by unemployment and found it more challenging to return to the labour market once the crisis was over. Furthermore, their duties often include caring for the sick (Kapur, 2020; Casale and Posel, 2021; Ba, 2020) and the household. Consequently, they turn away from productive work activities, which has consequences in terms of income.
In addition, girls may be compelled to reduce their time on schooling compared to boys to help their mothers with household chores (collecting water, for example). Moreover, health services are overwhelmed in crises such as the Ebola or Zika virus, and women have less access to pre-and post-natal care and contraceptives (Azcona et al., 2020). These and other non-economic effects could leave women in very precarious circumstances and have long-term repercussions on the vulnerability of women and girls, leaving them even further behind their male counterparts in terms of equality.
It is, therefore, essential to take the gender dimension into account when studying the effects of Covid-19 (Agarwal, 2022; Smith, 2019; Wenham et al., 2020), especially given that the gender dimension is often omitted in pandemic impact assessments. For example, according to Criado Perez (2019), less than one per cent of the 29 million scientific articles published concerning the impact of Zika and Ebola focused on gender.
Previous studies on the gender impacts of Covid-19 show that women are more affected than men in terms of employment (Alon et al., 2022; ILO, 2020; Casale and Posel, 2021) and poverty (Chitiga et al., 2022; Escalante and Maisonnave, 2022). In India, the gender gap on the labour market has reduced during 2020. It is due to a reduction in opportunities for men. At the same time, domestic chores have increased dramatically for women (Deshpande, 2022).
Indeed, the pandemic can accentuate existing inequalities and exposes vulnerabilities in health and labour market systems. For instance, informal workers do not benefit from safety nets when a lockdown is decided. Here, women are over-represented among informal workers.
The above gender issues are well illustrated in Senegal. Indeed, informal employment is the primary source of employment in Senegal, with 82% of the population working in the informal sector. However, this figure is higher for women (89%) than for men (76%), which indicates that women are more vulnerable in the labour market (ANSD, 2017). Regarding paid employment, 67% of women work in the service sector, 26% in agriculture and 5% in industry (World Bank, 2022). Furthermore, the data indicate that 73% of women work in vulnerable employment (World Bank, 2022). These are unpaid, family-worker jobs as well as self-employment jobs. Due to their instability, these jobs expose women to more precariousness.
Along with other countries, Senegal has been affected by the Covid-19 pandemic, especially in the Dakar region. Senegal has taken steps to halt the transmission chain of the virus (closure of schools, restriction on the movement of people). As in other countries, the general objective of these measures was to limit the proliferation of the virus and to restrict the number of people getting sick so as not to undermine the country’s health services. These measures have reduced working hours and the mobility of individuals and slowed the flow of transactions and, thus, the pace of economic activity. Figure 1, provided by Ridde and Faye (2022, p. 328), presents the number of Covid-19 cases in Senegal and the timing of the major Covid-19 between the first case and mid July 2022.
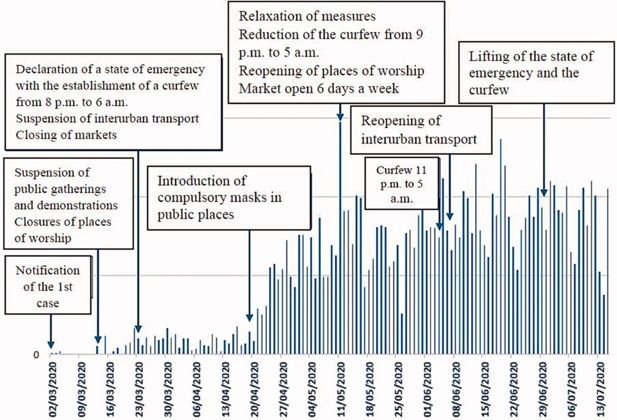
Number of Covid-19 cases and timing of Covid-19 response measures in Senegal in 2020 (Ridde and Faye, 2022, p. 38).
In this paper, we aim to evaluate the impact of Covid-19 on women so that gender-specific policies adapted to epidemics can be identified. To achieve this, we use a computable general equilibrium (CGE) model calibrated with the latest data available that considers the distribution of employment across both the formal and informal sectors of the economy and the gender dimension. Furthermore, we link the CGE model to a micro-simulation model to assess redistributive impacts. The remainder of the paper is organised as follows: section 2 presents the women’s situation in the labour market, section 3 describes the methodology and data used, and section 4 presents the scenarios and the results before the conclusion in section 5.
2. The situation of women in the labour market in Senegal
In Senegal, women comprise a relatively large percentage of the working-age population. The share of the working-age population among women is estimated at 80%, while for men, it is 75% (ANSD, 2017). The participation rate reveals gender disparities in labour market participation. Although the female working-age population is greater than that of men, the activity rate for men (60%) is about 20 points higher than that of women (40%) (ANSD, 2017). In addition, their marital status influences their likelihood of finding a job in the labour market (Adjamagbo et al., 2006).
Women predominate in some specific sectors, such as the manufacture of dairy products and ice cream (96% of the workforce is female) or the catering and drinking establishments sector, where 83% of the workforce is female. However, in all the other sectors, men predominate.
More than 80% of the women employed in the labour market are concentrated in five out of 21 economic sectors:1 "Growing of Cereals", "Trade and Repair" and "Other Services", "Growing of tubers and dry leguminous vegetables" and "Catering and drinking establishments". More than 80% are concentrated in relatively small sectors, increasing their labour market vulnerability. Regarding education, men systematically outnumber women, and this gap widens progressively as the educational level increases. While 47% of unskilled workers are women, only 23% of the highly skilled (with master’s or doctorate degrees) are women. Over 80% of women have no qualifications, and only 2.5% qualify as equal to or superior to the high school degree (Table 1).
Distribution according to gender and level of education (%) and the distribution of education among women.
Distribution among men and women | Level of education among women | |||
---|---|---|---|---|
Women % of total | Men % of total | Total | % of women | |
Without education | 47 | 53 | 100 | 86.2 |
Certificate of Primary Education (CEPE) | 44 | 56 | 100 | 7.4 |
Junior Secondary Education Certificate | 36 | 64 | 100 | 3.7 |
Secondary School-leaving certificate (BAC) | 30 | 70 | 100 | 1.6 |
General Undergraduate degree University Diploma / | 23 | 77 | 100 | 0.6 |
Degree/Master’s/Engineer/Doctorate | 23 | 77 | 100 | 0.5 |
Overall | 46 | 54 | 100 | 100 |
-
Source: ANSD (2017).
This gap in education level is reflected in the occupation of the labour market. Indeed, slightly more than 60% of the women are either "self-employed non-agricultural workers" or "family carers". Employment in both types of jobs requires few skills; therefore, income is low. Moreover, both types of employment are characterised by a high degree of insecurity. There are fewer women in senior management positions and as employers. Most are family carers or self-employed non-agricultural workers (ANSD, 2017). In addition, only 22% of permanent and 32% of fixed-term contracts are held by women, further accentuating their precariousness in the labour market.
3. Methodology and data
3.1. The Macro model and data used
To evaluate the impacts of Covid-19 and the induced lockdown on the Senegalese economy, we use the PEP 1-1 CGE model developed by Decaluwé et al. (2013). CGE models represent the different economic sectors, institutions and labour force, particularly the female labour force. These models enable the different impacts of Covid-19 (international and national impacts) to be captured and are, therefore, appropriate tools to use in this context. Moreover, they can capture shocks from the rest of the world and transmit them to the national economy, capturing impacts on households, businesses and governments.
CGE models consider inter-sectoral linkages while capturing the different transmission effects between sectors. Therefore, as previously mentioned, these models are the appropriate tools to analyse the direct and indirect impacts caused by the Covid-19 shock, as was the case for studies carried out by Maliszewska et al. (2020) and Laborde et al. (2020). Furthermore, these models have also been used to evaluate the impacts of previous pandemics (Beutels et al., 2009; Keogh-Brown et al., 2010). A few studies using CGE models to assess the impacts of Covid 19 on women exist (e.g., Chitiga et al., 2022; Escalante and Maisonnave, 2022).
Our model is calibrated on the latest Social Accounting Matrix (SAM) developed by Cabral (2020) using data from 2017. It highlights the duality of the Senegalese economy, divided into formal and informal compartments.2 Our model comprises 36 activities and 46 products in line with the SAM. Each sector of production uses labour, capital and intermediate consumption to produce. Our model distinguishes six different types of the labour force, broken down according to the level of skills (unskilled, semi-skilled and skilled) and gender (men, women). All activities use different types of labour but in varying proportions. For example, the formal highway sector uses relatively higher levels of unskilled male labour, while the informal crop sector uses relatively higher levels of unskilled female labour. In addition, the formal sectors combine labour with formal capital, while the informal sectors combine labour with informal capital.
Technically, the production function is a nested function with five levels. The output is a Leontief-type function between value added and intermediate consumption at the first level. The value added is a constant elasticity of substitution (CES)-type function between composite labour and capital. At the third level, composite labour is disaggregated according to the skills (skilled, medium-skilled and unskilled). At the last level, each type of work is a CES-type function between men and women.
We identify four different institutions in the model: households, firms, government and the rest of the world. Households are classified according to their income decile and whether they reside in Dakar, other urban centres (OUC) or rural areas. They derive their income from labour, capital and transfers. Households from Dakar mainly retrieve their income from labour (48%) and capital income (28%). Households from OUC get their income from labour (38%), capital income (32%) and transfers from the rest of the world (14%). Households living in rural areas mainly receive income from labour (42%) and transfers from abroad (15%). Households spend most of their income on final consumption, with the remainder allocated to paying direct taxes, transfers to other economic institutions and savings. Specifically, households living in Dakar spend 59% of their income on consumption spending, reaching 61% for households in OUC and 68% for rural households. The consumption basket of urban households (Dakar and other urban centres) comprises industrial products and market services. In contrast, rural households are mainly composed of industrial and agricultural products.
Firm’s income is derived from capital income and transfers from other agents. They distribute dividends to other agents, pay corporate taxes and save the rest.
The government’s income comes from capital income, direct and indirect taxes and transfers from other agents. From the SAM, we can see that the primary sources of income for the Senegalese government are indirect taxes (33%), capital income (20%), direct taxes (22%) and import duties (12%). The government uses its income mainly for non-market sector production (73%) and makes transfers to other institutions (19%). Public savings represents the difference between income and expenditure.
To link Senegal and the rest of the world, the traditional CGE modelling approach is used, where trade is modelled based on the assumption of imperfect substitutability of products given their origin, i.e., the Armington assumption (Armington, 1969). We assume that Senegalese producers can sell their products either on the local market or as exports on the international market. However, to increase their global market shares, Senegalese producers must be more competitive than international producers. Technically, it means that we assume a finite elasticity for the export demand that reflects the competitiveness of local producers in the international markets.
One of the transmission channels of the Covid-19 crisis stems from exchanges with the outside world. Consequently, we will outline the trade situation in Senegal.
The Senegalese economy is entirely dependent on the international market. Consequently, the import penetration rate is relatively higher than the export rate, contributing mainly to the structural nature of the trade balance deficit. Indeed, while imported goods and services account for nearly 21% of the domestic market supply (local sales and imports), slightly less than 14% of the total local production is sold on the international market (Table 2). The secondary sector is the leading exporter of goods and services (66% of total exports). Among this category, manufactured products, agro-food industry products, other extractive industrial products and refined petroleum represent 18%, 15%, 15% and 9% of total exports. Agricultural products play a very modest role in exports (only 8% of the total). Among the goods whose production is most oriented towards the international markets are mining products (80%), tobacco (65%) and oil (64%).
International trade
Share of Exports per commodity (%) a | Share of imports (%) b | Rate of export (%) c | Penetration rate (%) d | |
---|---|---|---|---|
Agriculture | 8.1 | 5.9 | 8.7 | 10.3 |
Industry | 65.8 | 84.2 | 23.4 | 39.0 |
Market services | 26.1 | 9.8 | 9.2 | 5.9 |
Non-market services | 0.0 | 0.0 | 0.0 | 0.0 |
Total | 100 | 100 | 13.7 | 20.6 |
-
Source: Computation from the authors based on the Social Accounting Matrix (SAM) 2017 (a) export by sector/total export, (b) Import by sector/total export, (c) Export by sector/Production by sector, (d) Import of commodity/ (imports + locally produced commodity).
On the other hand, industrial products make up the majority of total imports (84%). Among this category, we find commodities such as manufactured goods (43%), oil (11%) and products from other extractive industries (10%). Almost 40% of industries' commodities sold in Senegal are imported. Among this category, manufactured goods, oil and products of other extractive industries are the markets that rely the most on the rest of the world, where 81%, 78% and 66%, respectively, come from foreign producers.
In terms of closures, we assume that the nominal exchange rate is the numeraire of the model. Furthermore, we follow the small country hypothesis; consequently, world prices are exogenous.3 We also assume that the current account balance and government spending are fixed. Finally, we assume that labour and capital are mobile across sectors. Compared to the PEP model,4 we assume there is unemployment in the labour market. Indeed, Senegal has a high unemployment rate, particularly for women.
Consequently, we assume there is unemployment for skilled and medium-skilled workers following Blanchflower and Oswald (1995)5. This approach assumes an inverse relationship between wages and the unemployment rate. We assume a segmentation of workers between public and private workers. Indeed, public sector workers are guaranteed employment and are therefore not affected by labour market adjustments. We have not introduced any segmentation between the formal and informal sectors of the economy. There is a specific porosity between these two types of sectors in the sense that if a worker in the formal sector lost his job, he would seek to work in any branch, formal or informal.
3.2. The micro model – labour market
To assess the impact of Covid-19 on the employment of men and women, we build a micro-simulation model of jobs and link it to the CGE model. Indeed, using the National Employment Survey of Senegal from 2017 (ANSD, 2017), employed individuals can be matched, according to their qualifications, to each segment of the labour market and each sector. This survey makes it possible to identify the central occupational sector of the individual. Furthermore, this model allows us to simulate the impact of the Covid-19 shock on demand for workforce per skill category.
For each of the sectors, the workforce in the different segments of the labour market is given according to gender: skilled workers (bac + 4 years additional education and more), medium-skilled workers (bac + 2 years additional education or +3 years additional education, bac, BFEM/BEP, CFEE), and unskilled workers (uneducated or those who have not completed primary education). Each of the different labour market segments is then associated with the corresponding number of employees and sectors. For each market segment, we determine the number of employees involved. These employees are distributed across the different areas of activity according to their level of qualification and gender. From the simulation, we obtain employment volume variations by sector and category.
3.3. The micro model – poverty analysis and data
The distributive impact analysis of Covid-19 on households consists of two steps. First, we execute a micro-simulation. We use the top down non behavioural approach as presented in Cockburn et al. (2014) by transferring the information of the CGE model household survey data by the WAEMU (2018). As a second step, we execute a distributive analysis by computing inequality and poverty indicators and comparing their values between the scenarios. In Senegal, most households are male-headed (i.e., 76%), and poverty applies significantly less to female-headed households than to male-headed households. Therefore, we differentiate into rural and urban households, as it was making more sense for Senegal.
From the survey data (ANSD, 2021), we derive four subsamples representing the households in different regions (j): all households in Senegal (i.e., at the national level), urban households living in Dakar, other urban living in OUC, and rural households. Table 3 presents the descriptive statistics for the regional and the national sample. The difference between the regional and national samples indicate that —as expected— the households in Dakar regions have significantly higher consumption per head than all households at the national level. The households in other urban regions are, in terms of mean and median, relatively close to the national-level values, while households in the rural region have the lowest spending. These differences illustrate that at the microeconomic scale, the situation of spending/income is very different and requires differentiated analysis.
Descriptive statistics of the consumtion per head for the regional samples, values in Million CFA franc
Min. | 1st Quartile | Median | Mean | 3rd Quartile | Max. | |
---|---|---|---|---|---|---|
National | 0.051 | 0.279 | 0.428 | 0.652 | 0.717 | 16.123 |
Urban Dakar | 0.254 | 0.734 | 1.149 | 1.537 | 1.888 | 16.123 |
Urban Other | 0.068 | 0.337 | 0.498 | 0.666 | 0.756 | 14.332 |
Rural | 0.051 | 0.218 | 0.310 | 0.391 | 0.456 | 5.157 |
To simulate the economic changes at the household level, we apply the relative changes of the nominal total consumption budget (CTHi,j) per household to the total consumption per household provided by ANSD (2021) (e.g. dtoti,j). However, first, we standardise the total household spending by dividing them by the number of household members (hhsizei,j) and weight them by the spatial deflator (def_spai) provided by ANSD (2021).
With i: household type according to the households income decile j: regional of households residency: urban in Dakar region, urban in other regions, rural
We define the poverty line according to the value of the poverty headcount index (Equation 3) for the four regional subsamples according to ANSD (2021) and simulate the change of the poverty line by multiplying with the change of the consumer price index (PIXCON) (Equation 4).
Based on the simulated values for the spendings (dtotSCEN,i,j) and poverty line (zrefSCEN,i), we compute the values for inequality and poverty as Gini-index (Gini, 1936) for and as the Foster-Geer-Thorbecke (Foster et al., 1984) indexes by using the software package “ineq” (Zeileis, 2014).
4. Scenarios and simulation results
4.1. The identified channels
The Covid-19 pandemic affects Senegal through external channels and channels specific to the Senegalese economy. On the one hand, the country is affected by the reduced economic activity of its main trade partners. Therefore, Senegalese exports are expected to decrease, given the decline in economic activity, especially in China. However, given that Senegal’s main trading partners are the countries of the sub-region, this decline is relatively moderate.
Looking at the year-on-year change in exports between October 2019 and October 2020, export demand declines for some products while it increases for others (see Table 4). The products most affected are hospitality, telecommunications services and fishery products (ANSD, 2020a). Moreover, world price changes have been relatively limited and specific to certain products in the same period (agri-food commodities, manufactured products and peanut oil).
Simulated scenarios
Moderate Scenario | Severe Scenario | |
---|---|---|
International channels | ||
Decline in exports | Fishing and aquaculture production: - 52.5% Agro-food production: -8.4% Petroleum refinery products: -15% Chemical products: -22.9% Telecommunications services: -59% Air transport services: -30% Hospitality services: -78% Catering services: -20% | |
Increase in exports | Agricultural and associated products: +6.2% Livestock products: +92.6% Extractive industry products: +5% Manufacturing industry products: +32.4% | |
Lower international prices | Peanut oil: -8.2% Agri-food products: -2.6% Non-food industry products: -2.6% | |
Decline in migrant remittances | -10% | -15% |
Domestic channel | ||
Declining productivity of sectors | highly affected sectors: -2% | highly affected sectors: - 3% |
moderately affected sectors: -1% | moderately affected sectors: -2% |
Furthermore, remittances will be another external channel through which the Senegalese economy will be affected. Senegalese households receive remittances from family members living outside the country. These remittances accounted for 10% of GDP in 2018. Most of these come from France, Italy and Spain. These countries are struggling economically due to the Covid-19 pandemic. The Central Bank of West African States (BCEAO) believes these remittances may decline by 30% in 2020.6
Alongside these external shocks, the Senegalese government has also taken measures to restrict the movement of people, and closing businesses and borders have also affected the Senegalese economy. Therefore, these restrictions will affect all sectors of the economy in 2020, but to different extents. Indeed, a representative study carried out in the industrial sector reveals that 93% of companies have been affected by Covid-19 (ANSD, 2020b). For most businesses, the health crisis has resulted in a decline in production by more than 25%. In comparison, 15% believe their productive activity has declined by more than 75%. In addition, business leaders claim that government-imposed restrictions on worker mobility due to curfews significantly reduce worker productivity (ANSD, 2020b).
The sectors most affected are the tourism sectors (hotels and restaurants), due to the suspension of international flights and the cancellation of cultural events such as the Dakar Biennale, and the transport sectors, due to the measures restricting the movement of the people and curfews. According to the business leaders interviewed, this temporary cessation of business activity was mainly due to the closure of borders, markets, schools, and universities, requiring parents to stay at home (ANSD, 2020b).
In order to consider this decline in production brought about by the closure of stores/companies and the decline in the number of hours worked, we suppose the loss of productivity will vary from sector to sector depending on the degree to which they are impacted. Therefore, we have distinguished three categories of sectors: those which are highly affected, such as tourism and transport; those which are moderately affected and those which are not affected at all, such as the agro-food industry.
It is rather challenging to quantify the impacts of closures and declining labour productivity. Therefore, we suggest an evaluation using a "moderate" scenario and a "severe" scenario. These two scenarios differ in their level of severity. The first “moderate” scenario assumes that the Senegalese economy returns to a pre-Covid situation relatively quickly and that the decline in productivity does not overly impact the sectors. On the other hand, the "severe" scenario assumes that the social distancing and “work at home” measures will significantly impact the sectors' productivity. Moreover, we assume that remittances from migrants decrease more in the severe scenario than in the moderate scenario.
While the magnitude of the shocks in international prices, export quantities, and transfers are documented, the decline in sectoral productivity is not based on any estimates, as precise data is unavailable. Our objective is to provide a reasonable estimate. Increasing the magnitude of the decline in productivity will likely produce more severe results.
4.2. The results (Macro-economic impacts)
4.2.1. Impact on macroeconomic variables
The macroeconomic impacts of the Covid-19 pandemic are considerable, with the economy being simultaneously affected on both the demand and supply sides. On the supply side, workers are less productive, and capital is less used. Thus, production is declining in most sectors. This combined effect leads to a 5.4% reduction in the gross domestic product (GDP) for the moderate scenario and a 6.5% reduction in GDP in the severe scenario (Table 5). The decline in total production also leads to a decline in the total demand for labour in each firm, which increases the unemployment rates for each labour category. The total labour demand for men declines by 0.8%, while women’s labour demand declines by 0.7%. Increasing unemployment and declining household wage rates lead to decreased incomes and consumption.
Impact on macroeconomic variables (% change)
Moderate | Severe | |
---|---|---|
Real GDP | -5.4 | -6.5 |
Total investment | -7.0 | -8.8 |
Consumer Price Index | -2.9 | -3.1 |
Total employment | ||
Male | -0.8 | -1.0 |
Female | -0.7 | -0.8 |
Real consumption of households located in | ||
Dakar | -2.7 | -4.0 |
urban regions | -3.2 | -5.0 |
rural areas | -3.3 | -5.1 |
-
Source: CGE model results.
4.2.2. Sectoral impacts
Most sectors face a decline in production caused by declining factor productivity, while some face a decline in external demand. Thus, the impacts on sectors differ depending on the impacts caused by lockdown and social distancing measures (e.g., reduced labour productivity) and international trade (i.e., reduced export demand). For example, the hotel and catering sector faces a decline in productivity and an extreme decline in international demand from tourists. Thus, production falls sharply in both scenarios, and these sectors reduce the demand for labour (by 31%) and intermediate consumption, which affects other sectors.
For the construction sector, the decline in production is induced partly by the drop in labour productivity and mainly by the decline in the total investment budget. As a result, this sector sees its production decrease by 28% in the moderate and 31% in the severe scenario.
The agro-food industry increases in production, given the increase in export demand. In addition, the crop sector benefits from increased foreign demand for agricultural and livestock products (see Table 4), such as cereals, fruit, and vegetables. For the industry to produce more, it will recruit workers. Recruitment will be cheaper as the availability of workers made redundant from the other sectors increases.
4.2.3. Impact on male and female employment
Overall, the effect on employment is negative. The decline in employment is more significant for men (-0.8%) than for women (-0.7%), irrespective of the scenario. Looking at the impacts by employment type, skilled women are relatively more affected than skilled men, with an unemployment rate that increases by 2.7 percentage points (p.p.) for women compared to 2.6 p.p. for men in the moderate scenario and by 3.7 p.p. and 3.5 p.p. for women and men respectively in the severe scenario.
On the other hand, the unemployment rate for medium-skilled workers increases by 2.1 p.p. for men, compared to 1.8 p.p. for women and by 2.9 p.p. and 2.5 p.p. for men and women, respectively, in the severe scenario. Women are relatively less affected than men in the labour market because the recruiting sectors, given the increase in external demand, tend to be relatively more female labour-intensive. For example, it is the case for the dairy and agricultural products industries.
Given the general slowdown in activity, sectors are reducing the number of employees, and wage rates are declining. Thus, the wage rate for skilled men declines by 4.3% compared to 3.9% for women, with the rate for medium-skilled men declining by 4.4% and by 3.9% for women, while the wage rate for unskilled workers is the most affected, declining by 5.9% for men and by 5.2% for women. Moreover, the general decline in activity leads to a decrease in the return on capital by about 5.9% in the moderate scenario and by 7% in the severe scenario.
To more accurately analyse the labour market, we can determine the changes to the workforce using a microsimulation model. For example, the Covid-19 shock led to the destruction of 54,355 jobs in the moderate scenario compared to 66,242 jobs in the severe scenario.
The destruction of jobs in both scenarios is more significant in the informal sector than in the formal one. There are four times more job losses in the informal sector for the moderate scenario (Table 6). As shown above, the formal sectors benefit from an increase in foreign demand, which offsets the adverse effects of Covid-19. The informal sectors do not benefit from this lever and therefore incur the full adverse effects of Covid-19.
Change in the number of employees per segment of the economy (No. of workers)
Moderate | Severe | Moderate | Severe | |
---|---|---|---|---|
absolute change | relative changea | |||
Formal sector | -9,866 | -11,960 | -0.3% | -0.3% |
Informal sector | -44,489 | -54,282 | -1.2% | -1.5% |
Total | -54,355 | -66,242 | -1.5% | -1.8% |
-
Source: results of the micro model.
-
(a) Referred to the total number of employees of 3,712,719.
The sectors that make the most redundancies are "accommodation and catering" (-30,593 jobs), "other non-food industries" (-17,250 jobs) and "trade and repair" (-15,051 jobs). Men, as indicated earlier, experience more job losses. While in the moderate scenario, the loss of jobs is relatively close between men and women, the gap widens in the severe scenario (Figure 2). Such job losses are mainly recorded amongst the unskilled worker category, which sees a loss of 53,615 jobs in the severe scenario and 43,840 jobs in the moderate scenario. Skilled workers (both men and women) are relatively unaffected by job losses compared to the other two categories of workers.
4.2.4. Impact on agents
Given the decrease in wages and the drop in employment, all households experience a decrease in revenue, on average, by 6% in the moderate and 7.5% in the severe scenarios. However, the loss of income is not homogeneous among the different categories of households. Indeed, households face a decline in factor remuneration (capital and labour) and a decrease in remittances from abroad. In rural households and households living in other urban centres, remittances account for up to 14% of the total income (see section 2.1). Consequently, the remittance decline will most affect these two types of households. With declining incomes, households consume less, reduce their savings and pay less direct taxes to the government.
Public administration revenues decline because of lower direct taxes paid by households and businesses and lower indirect tax revenues. Public administration revenues decline by 5.0% in the moderate scenario and by 6.2% in the severe scenario. This decline in government revenues and fixed expenditures leads to a massive increase in the government’s current account deficit (-72% for the moderate scenario and -93% for the severe scenario). The decline in the income and savings of all agents leads to a decrease in the total investment budget (see Table 5), impacting the construction and machinery sectors.
4.3. Impacts on inequality and poverty
Figure 3 compares the change in indicators, which measure inequality and poverty. In the scenarios, the inequality changes only marginally because the relative changes in consumption are in the same direction for the households and a close range within income groups. The number of households falling poor increases in all regions in both scenarios, especially in the severe scenario. Between 2011 and 2018, poverty decreased by five percentage points at the national level. The Covid-19 pandemic has set back the positive development to reduce poverty between 2011 and 2018.
The most significant increase in poverty applies to urban households not located in the Dakar region. Here increases in prices and their impact on non-agricultural jobs are particularly relevant. Rural households are also high in the poverty gap and poverty severity. Thus, in rural regions, poverty worsens in all dimensions since the income level is already shallow and poverty indicators are very high. Urban households in the Dakar region are the least impacted households since, here, the income level is sufficiently high to cope with increased prices.
5. Conclusion and policy recommendations
This study provides information on the impact of Covid-19 on the Senegalese economy with a focus on women’s employment and poverty. To capture the different impacts of Covid-19 (national and international), a CGE model was used, along with a microsimulation module, to obtain a more detailed picture of the impact on employment and poverty. The Senegalese economy suffered from the measures taken to contain the Covid-19 epidemic, with a 5% decrease in GDP under the moderate scenario. While most sectors of the economy are affected, those with increasing external demand (e.g., agriculture) have fared better. In terms of employment, unskilled workers are the most impacted group. Women, although strongly impacted, tend to be less affected than their male counterparts. This is due to the predominant presence of women in the agricultural sectors, which are positively impacted by the increase in international demand for agricultural products. In terms of poverty, the pandemic has halved the progress made between 2011 and 2018. The increase in poverty incidence is exceptionally high in rural and urban households outside Dakar. Here, the drop in remittances increases the vulnerability of these households.
It is essential to point out that this work includes neither any government support policy nor the domestic work carried out by women and men, even though we know that women carry this workload more than men. Including this latter hypothesis in our model would likely cause women’s welfare to deteriorate further. Given the very harmful impact of the epidemic on some sectors, the government should give special consideration to the workers in these sectors. Furthermore, pro-poor policies could be regionally targeted to help households outside Dakar and rural households.
The present study was executed in the year 2020 in the mid of the Covid-19 crises as early ex-ante impact assessment. At this time statistical data were not available and expectations of the economic development were more pessimistic than reality had showed later. For instance, the development of remittances was simulated as decrease by 10% and 15%, while remittances increased by 2%; the development of GDP was simulated as decrease by 5% and 7%, while in reality GDP increased by 1%. Interpretation of the study’s result require considering the worse-than-reality-assumptions of the simulated scenarios.
Footnotes
1.
The sectors comprise three primary sectors, eight secondary sectors and nine service sectors; 15 out of the 21 sectors are differentiated in formal and informal sectors.
2.
Note that the sectors are not modeled differently as in Fortin et al. (1997) but just separate sectors not related to each other producing the same good.
3.
With these hypotheses, we assume that Senegal cannot influence world prices. Senegalese producers are price takers. Assuming that the current account balance is fixed means that Senegal cannot borrow from the rest of the world.
4.
Note that compared to the PEP standard model, we have changed the assumption on the labour market and the nested functions for the value added.
5.
This approach to model unemployment is quite common. See for instance Decaluwé et al. (2005)
6.
Ex-post, however, World Bank (2022) estimates an increase of remittances by 2% in Senegal from 2019 to 2020 and a decrease by 16% and 14% in Western and Central Africa and in Sub-Sahara Africa (World Bank, 2022). Thus, the development simulated in this study based on ex-ante estimations were too pessimistic.
References
-
1
Inequality in the impact of the coronavirus shock: Evidence from real time surveysJournal of Public Economics 189:104245.https://doi.org/10.1016/j.jpubeco.2020.104245
-
2
Comment les femmes concilient- elles mariage et travail à Dakar et à Lomé? Working Papers DT/2006/04, DIAL (Développement, Institutions et Mondialisation)1–18.
-
3
Imperatives of recognising the complexities: gendered impacts and responses to COVID-19 in IndiaEconomia Politica (Bologna, Italy) 39:31–53.https://doi.org/10.1007/s40888-021-00242-8
-
4
Gendered Impacts of COVID-19 in Developing CountriesAEA Papers and Proceedings 112:272–276.https://doi.org/10.1257/pandp.20221013
-
5
Enquête Nationale Sur l’Emploi Au SénégalDakar, Sénégal: Agence Nationale de la Statistique et la Démographie (ANSD).
-
6
Données Sur Les Variations Des ExportationsDakar, Sénégal: Agence Nationale de la Statistique et la Démographie (ANSD).
-
7
Impact COVID-19 Dans l’industrie. Enquête COVID-19 Industrie (ECI)Dakar, Sénégal: Agence Nationale de la Statistique et la Démographie (ANSD).
-
8
Enquête Harmonisée Sur Les Conditions de Vie Des Ménages (EHCVM) Au Sénégal – Rapport FinalDakar, Sénégal: Agence Nationale de la Statistique et de la Démographie (ANSD).
-
9
A Theory of Demand for Products Distinguished by Place of ProductionStaff Papers (International Monetary Fund) 16:159–178.
-
10
From Insights to Action: Gender Equality in the Wake of COVID-19. UN WomenFrom Insights to Action: Gender Equality in the Wake of COVID-19. UN Women, https://www.unwomen.org/sites/default/files/Headquarters/Attachments/Sections/Library/Publications/2020/Gender-equality-in-the-wake-of-COVID-19-en.pdf.
- 11
-
12
The economic impact of SARS in Beijing, ChinaTropical Medicine & International Health 14 Suppl 1:85–91.https://doi.org/10.1111/j.1365-3156.2008.02210.x
-
13
An Introduction to the Wage CurveJournal of Economic Perspectives 9:153–167.https://doi.org/10.1257/jep.9.3.153
-
14
The indirect impact of COVID-19 on womenThe Lancet. Infectious Diseases 20:904–905.https://doi.org/10.1016/S1473-3099(20)30568-5
- 15
-
16
Gender inequality and the COVID-19 crisis: Evidence from a large national survey during South Africa’s lockdownResearch in Social Stratification and Mobility 71:100569.https://doi.org/10.1016/j.rssm.2020.100569
-
17
How COVID-19 Pandemic Worsens the Economic Situation of Women in South AfricaThe European Journal of Development Research 34:1627–1644.https://doi.org/10.1057/s41287-021-00441-w
-
18
Handbook of Microsimulation Modelling, Contributions to Economic Analysis275–304, Macro-Micro Models, Handbook of Microsimulation Modelling, Contributions to Economic Analysis, Vol, 293, Emerald Group Publishing Limited, p.
-
19
Invisible Women: Data Bias in a World Designed for Men432, Invisible Women: Data Bias in a World Designed for Men, New York, Abrams Books, p.
- 20
- 21
-
22
The Covid-19 pandemic and gendered division of paid work, domestic chores and leisure: evidence from India’s first waveEconomia Politica (Bologna, Italy) 39:75–100.https://doi.org/10.1007/s40888-021-00235-7
-
23
Falling living standards during the COVID-19 crisis: Quantitative evidence from nine developing countriesScience Advances 7:eabe0997.https://doi.org/10.1126/sciadv.abe0997
-
24
Gender and Covid‐19: Are women bearing the brunt? A case study for BoliviaJournal of International Development 34:754–770.https://doi.org/10.1002/jid.3603
-
25
Taxation, wage controls and the informal sectorJournal of Public Economics 66:293–312.https://doi.org/10.1016/S0047-2727(97)00013-3
- 26
-
27
On the Measure of Concentration with Special Reference to Income and Statistics, General Series No. 20873–79, On the Measure of Concentration with Special Reference to Income and Statistics, General Series No. 208, Colorado College Publication, p.
- 28
-
29
Gender Analysis: Prevention and Response to Ebola Virus Disease in the Democratic Republic of Congo.] CAREhttps://www.care- international.org/files/files/Ebola_Gender_Analysis_English_v2.pdf, Accessed, 1 May 2020.
-
30
The possible macroeconomic impact on the UK of an influenza pandemicHealth Economics 19:1345–1360.https://doi.org/10.1002/hec.1554
-
31
COVID-19 and Global Food Security, Part One: Food Security, Poverty, and Inequality16–19, Poverty and food insecurity could grow dramatically as COVID-19 spreads, COVID-19 and Global Food Security, Part One: Food Security, Poverty, and Inequality, Washington, DC, International Food Policy Research Institute (IFPRI), p, 10.2499/p15738coll2.133762_02.
-
32
The Potential Impact of COVID-19 on GDP and Trade: A Preliminary AssessmentThe Potential Impact of COVID-19 on GDP and Trade: A Preliminary Assessment, Policy Research Working Paper 9211, The-Potential-Impact-of-COVID-19-on-GDP-and-Trade-A-Preliminary-Assessment.pdf.
- 33
- 34
-
35
Policy response to COVID-19 in Senegal: power, politics, and the choice of policy instrumentsPolicy Design and Practice 5:326–345.https://doi.org/10.1080/25741292.2022.2068400
-
36
Overcoming the ‘tyranny of the urgent’: integrating gender into disease outbreak preparedness and responseGender & Development 27:355–369.https://doi.org/10.1080/13552074.2019.1615288
-
37
Harmonized Survey on Households Living Standards, Senegal 2018-2019Ref. SEN_2018_EHCVM_v02_M.
-
38
COVID-19: the gendered impacts of the outbreakThe Lancet 395:846–848.https://doi.org/10.1016/S0140-6736(20)30526-2
-
39
The Impact of COVID-19 Pandemic on Women: Lessons from the Ebola Outbreak in W/AfricaAccra, Ghana: WANEP.
- 40
- 41
-
42
_ineq: Measuring Inequality, Concentration, and Poverty__ineq: Measuring Inequality, Concentration, and Poverty_, R package version 0.2-13, https://CRAN.R-project.org/package=ineq.
Article and author information
Author details
Funding
This work was carried out with financial and scientific support from the Partnership for Economic Policy (PEP), with funding from the Government of Canada through the International Development Research Centre (IDRC).
Acknowledgements
The authors thank two anonymous reviewers for detailed and constructive comments on the original submission to the International Journal of Microsimulation.
Publication history
- Version of Record published: December 31, 2022 (version 1)
Copyright
© 2022, Maisonnave et al.
This article is distributed under the terms of the Creative Commons Attribution License, which permits unrestricted use and redistribution provided that the original author and source are credited.