
Simulations of Policy Responses and Interventions to Promote Inclusive Adaptation to and Recovery from the COVID-19 Crisis in Ghana
Abstract
We assess the impact of COVID-19 shocks on household welfare and the effectiveness of select policies implemented to reduce their impact on welfare in Ghana. We adopt a microsimulation approach to assess the effects of COVID-19 on household welfare. Welfare fell by 34.2% to 41.9% between March and June 2020. Over the same period, the poverty headcount and the Gini index increased by 9 to 10.5 percentage points and 0.4 to 0.6 points respectively. The number of poor people increased by 2.8 to 3.2 million. The hardest-hit sector was education, with agriculture, forestry and fishing, trade and repairs, manufacturing, and other services also affected. The effects vary for men, women and children. While women experienced the largest decline in welfare, men experienced the highest increase in poverty incidence. The three policies selected reduced poverty marginally but were unable to offset the increase in poverty that occurred between March and June. The estimated cost of the three policies is GHS3.7 billion excluding administrative costs, which equates to approximately 1% of 2020 GDP.
1. Introduction
It has been over two years since Ghana experienced its first lockdown due to the COVID-19 pandemic. Even as countries recover from the pandemic, its effects are still felt around the world. The COVID-19 pandemic affected almost every sector of the economy, from GDP to poverty and unemployment (Arezki et al., 2020; Maliszewska et al., 2020; OECD, 2020; World Bank, 2020). It is estimated that the number of poor people could increase by 420 to 580 million globally, thereby derailing countries’ ability to achieve the sustainable development goal of no poverty (Sumner et al., 2020).
The pandemic’s effect at the household level has not been uniform. World Bank (2020) reports that the extent of the damage a household suffers is dependent on some household-specific demographic and socio-economic characteristics. While this is the case, Ataguba (2020) reports that, given demographic and socio-economic characteristics, poor and vulnerable members of society are likely to be more affected by the pandemic than rich households.
The Ghana Statistical Service (GSS) reported that households suffered welfare losses due to lost income because of layoffs and job losses. GSS estimates that 77.4% of households in Ghana suffered income losses due to COVID-19 lockdown restrictions. A similar percentage of households experienced difficulty purchasing food due to increased food prices.1 Households’ income losses will make it difficult for them to afford their usual basket of goods. This implies it will also be difficult to pay for food, utilities, education, health care, and household members’ other needs.
Job losses and business closures are contributing factors to the losses suffered by households in Ghana. GSS estimates that approximately 115,000 businesses closed either temporarily or permanently and that businesses’ sales decreased by 26% to 36% year-on-year in March and April 2020. The reduction in sales and input shortages affected most businesses’ operations and especially those of credit-constrained micro, small and medium-sized enterprises (MSMEs) in the formal and informal sectors. They limited businesses’ ability to meet their obligations to their workers and increase production and sales. Hotels and restaurants (and more generally, the tourism sector) were severely affected by closures and restrictions.
This paper aims to analyse (a) the impact of COVID-19 shocks on household welfare, and (b) the effectiveness of governmental programs implemented to reduce the pandemic’s impact on welfare in Ghana. It helps us to answer the following questions: First, to what extent did the pandemic increase poverty in Ghana, and did the regions that did not experience a lockdown suffer the same impact on poverty and inequality outcomes? Second, were the effects on welfare the same for men, women and children? And third, were the policies implemented gender-equitable and sufficient to reduce the pandemic’s impact on welfare? We focus on interventions implemented in the health and utilities sectors.
We simulate the following policies that aimed to reduce the pandemic’s impact on households:
Free electricity for lifeline consumers, and free water for all households
COVID-19 loans for the creative arts industry
Tax relief and allowances (50% of basic salary) for frontline health workers
We also consider one policy that was proposed but rejected during a parliamentary debate in 2020: for the government to absorb students’ fees to attend public universities. We therefore also assess the potential impact of the government absorbing 50% of the fees of public university students.
We contribute to the literature by assessing the COVID-19 pandemic in Ghana and some of the policies used to mitigate its impact. Additionally, we quantify the pandemic’s impact on poverty and inequality. Furthermore, we contribute by (a) simulating the impact of three policies implemented by the government (and one policy that was proposed but not pursued) to determine the pandemic’s impact on the population of Ghana, (b) comparing policy options and determining which were efficient and cost effective at mitigating the pandemic’s effects, and (c) providing gendered results to show the effects for men, women and children.
Our work differs from that of Aduhene and Osei-Assibey (2021), Bukari et al. (2021), Nkrumah et al. (2021) and Schotte et al. (2021) in terms of the methods used and the additional gender dimension of our analysis. Bukari et al. (2021) apply econometric tools to estimate COVID-19s impact on poverty and welfare in Ghana. They observe a decline in welfare and an increase in poverty that was relatively more severe for female-headed households and rural households. However, households in the middle and upper quantiles were the least affected by the pandemic. Schotte et al. (2021) use the difference-in-differences framework to estimate the lockdown’s impact on employment, working hours and earnings in Ghana. They find that informal workers suffered relatively more from the lockdown, and women and self-employed individuals saw a decline in their earnings due to the lockdown. Aduhene and Osei-Assibey (2021) approach their analysis using discourse analysis to provide the pandemic’s impact on households in Ghana. Finally, Nkrumah et al. (2021) draw from consumer demand theory to simulate the effect of the water and electricity subsidies on households. Their results show that the subsidies were regressive and therefore benefitted the poor relatively less than the richer segments of society.
We consider our work to be an extension thereof addressing the pandemic’s impact on women and children beyond the sex of the household head. Our paper’s weakness lies in the assumptions made regarding changes in remittances and income across sectors and updates made to the Ghana Living Standards Survey (GLSS) based on information about population growth and price increases from 2017 to 2020. Despite our assumptions, our results can be considered the lower bound for the pandemic’s potential impact on household welfare, and the results do inform us of the direction of the change in welfare outcomes, which is qualitatively consistent with the results of the previously mentioned papers.
In the next sections, we briefly review the literature, describe the conceptual framework and methodological approach used for our microsimulation, present our results and conclude the paper.
2. Background and brief literature review
Ghana, like most nations, instituted various containment measures and interventions to stop the spread of COVID-19, save lives, protect livelihoods, and ensure economic recovery amid the COVID-19 pandemic. Since Ghana recorded its first two confirmed cases on March 12, 2020, the various containment measures put in place by the Government of Ghana, as announced by the President in his first national address include: social distancing measures; travel restrictions; the compulsory wearing of “nose masks” (also known as face masks); enhanced testing; contact tracing and further testing; the suspension of all public gatherings, which resulted in the closure of all schools and universities; and mandatory quarantine (for at least 14 days) for all people arriving by air on the night all borders closed. After the four-week period of partial lockdown in the Greater Accra and Ashanti regions and Kasoa Township, the government started gradually easing restrictions, with public gatherings again being allowed, initially for a maximum of 25 people, and later, 100 people.
While these measures were essential to reduce the spread of the virus, their effects on people’s livelihood and the economy cannot be underestimated. Given that most of the Ghanaian working population is in the informal sector, the economic implications of the lockdown measures on this subset of the population remained a major challenge and forced the government to lift lockdown restrictions even when the number of infected cases was increasing. The easing of lockdown measures despite a surge in infected cases at the time was a common phenomenon, as the poor and vulnerable people were more likely to die out of hunger than the virus itself. Thus, the large informal sector, in Ghana, and the many daily wage earners, who have little or no savings, made it very difficult for the government to enforce a total lockdown of the country. This is because, for the many daily wage earners, a total lockdown implies no work and hence no income and no access to food or other household necessities. More importantly, the informal sector often consists of small and informal businesses, which are a source of sustenance and livelihoods for many, particularly the poor (Gondwe, 2020). In lieu of the loss of work and income, the Government of Ghana instituted the provision of free water for household and commercial use, cooked and uncooked food, and tax holidays, among other economic recovery measures, to lessen the impact of the lockdown on citizens (Ozili, 2022).
Gentilini et al. (2020) summarise the social assistance interventions implemented in Ghana: (a) food vouchers for about 400,000 people in locked-down areas of the Greater Accra and Ashanti regions and Kasoa township; (b) access to food at school for students allowed to return to high school and junior high school for their final exams; (c) the removal of mobile money transfer fees; (d) the payment of consumers’ water and electricity bills; (e) the waiving of taxes for health workers; and (f) the provision of GHS500,000 by the Social Security and National Insurance Trust (SSNIT) for COVID-19 relief efforts.
The pandemic’s economic and social effects are well documented and, as suggested by the World Bank, not short-term. They continue to be felt around the world. The economic disruptions include a decline in export revenues, remittances, foreign direct investment and tourism (Gentilini et al., 2020; World Bank, 2020)
A significant number of studies have been published to help us understand the pandemic and its effects. One strand of the literature investigates the impact of lockdowns. For instance, Abdelkhalek et al. (2020) provide evidence that a two-month lockdown could directly lead to an increase in poverty (of 4.5 percentage points (p. p) in Tunisia and 6 p. p in Morocco) and in inequality in the short-run. Lustig et al. (2020) provide evidence for Argentina, Brazil, Colombia and Mexico. Their microsimulation shows that the lockdown had a severe impact on poverty.
Some studies have also suggested that mitigation measures can reduce the impact of lockdowns and the pandemic on the poor (Lustig et al., 2020; World Bank, 2020).
Another strand of the literature suggests that COVID-19’s impact was heterogeneous, affecting people differently depending on their sex and age (International Monetary Fund, 2020; United Nations Economic Commission for Africa, 2020; United Nations Organization, 2020; World Trade Organization, 2020). International Monetary Fund (2020) reports the lockdown’s impact was not proportionate for men and women in Spain, Italy and Portugal. As the International Monetary Fund (2020) alluded to, the lockdown coincided with the closure of schools and had a greater impact on women than men since more women were employed in the education sector and provided childcare services. Similarly, United Nations Organization (2020) found that close to 300 million people globally lost their jobs and livelihoods, with women and children being the most affected. Similar findings have also been reported by United Nations Economic Commission for Africa (2020)—with the explanation that women and youth normally occupy lower ranks such as temporary and part-time jobs, which made them vulnerable to job loss during the pandemic. Abdelkhalek et al. (2020) also arrived at qualitatively similar results for Tunisia and Morocco.
Dang and Viet Nguyen (2021) show that gender-based differences in terms of permanent job and income losses exist not only in Ghana, but also in other countries. However, they did not find significant gender differences for temporary job losses. Meanwhile permanent job losses were more pronounced in China, Italy and the United States than Japan, South Korea and the UK (Dang and Viet Nguyen, 2021). Similarly, Hossain (2021) found significant gender differences in Ethiopia and India, and comparatively less significant ones in Peru and Viet Nam due to women having access to formal employment. Extensions beyond gender differences, for example, by Ranchhod and Daniels (2021) to include education level, age, race and geography, and Witteveen (2020) to include race and income class, indicate that minorities within the various demographic groups considered are relatively more affected by job losses.
In terms of age, the International Monetary Fund (2020) found that the lockdown in Italy, Spain and Portugal had stronger adverse effects on those aged 25 to 44 than those aged 45 to 65. The effects were relatively weaker for those over 65 years old. The explanation provided is that the younger generation relies strongly on current income for consumption, and thus, not working due to the lockdown reduced their access to current income. The older and retired individuals, on the other hand, could rely on their retirement income when they stayed at home during the lockdown. For Abdelkhalek et al. (2020), the lockdown had a larger and more significant negative effect on the welfare of children than adults.
The pandemic’s effects across various sectors of the economy must also be emphasised. United Nations Organization (2020) and Abdelkhalek et al. (2020) note that SMEs and the informal, tourism and hospitality sectors were the most affected. Furthermore, World Trade Organization (2020) notes that, apart from informal jobs, jobs that require face-to-face interaction, such as retail, education and care service jobs, were strongly affected by the lockdown measures. United Nations Economic Commission for Africa (2020) also found Southern African countries saw similar sectoral impacts as well as an impacts in the transport sector.
3. Conceptual Framework
COVID-19 is expected to generate a variety of shocks. For instance, a reduction in labour supply, an increase in prices and a decrease in remittances may occur, as discussed in the literature review. Shocks can affect households in different ways depending on their onset, duration and impact. According to World Bank (2020) the pandemic’s impact on households includes:
The direct effect of lost earnings
An indirect effect on earnings and employment due to decreased demand, and production and supply disruptions
A decrease in both international and domestic remittances due to the global nature of the pandemic
Price gouging due to an inadequate supply of household necessities and basic goods
A potential increase in health care costs
A disruption to schooling and school-related programmes, including school meal programmes
The potential effect of the focus on COVID-19 reducing the availability of care for other medical emergencies
Figure 1 shows the different channels through which the pandemic can impact welfare. In this paper, we focus on the ones that are most important in the Ghanaian context. Specifically, we focus on how the loss of earning, remittances and price changes due to the COVID-19 pandemic affected household welfare—and assess the policies implemented by government to mitigate adverse impacts on poverty and welfare.
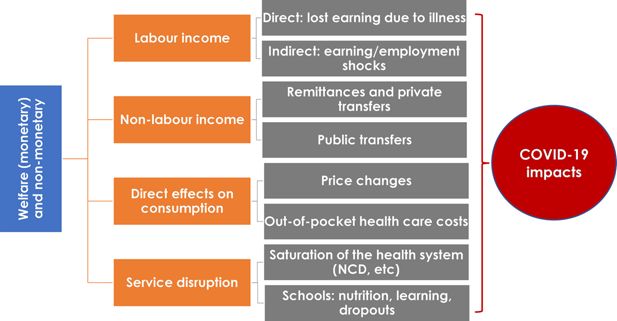
The channels through which COVID-19 impacts welfare in the short term. Source: Gentilini et al. (2020, p. 3), Figure 1.
4. Methodology
Formally, we assume that the impact of COVID-19 shocks on welfare is approximated by aggregating the effects on the main impact channels. If we denote the welfare of household at time by , the change in welfare due to COVID-19 shocks is:
where denotes the change in the welfare of household between period (pre-COVID-19) and (after COVID-19). The variable is the change in factor between and , and is the function linking factor to welfare. We use per adult equivalent expenditures as the proxy for welfare. The factors that are expected to affect household welfare in Ghana are remittances, labour income (distinguished by economic sector, source, etc.), public transfers, and consumer food prices.
To estimate the impact of (consumer) price variation on welfare, we use the first-order Taylor series approximation, namely,
where represents the expenditures of household on the food items in the consumers basket.
The decrease in labour income due to COVID-19 shocks depends on:
How vulnerable the sector or job in question is (for example, the tourism sector, or the trade and retail sector, which includes many informal jobs)
The amount of income earned by active household members
The duration and intensity of income shocks
Total household income equals the sum of the income or revenues of all economically active members. Total household income for household at time is:
where is the average income of the household in sector at time and is the number of household members that are active in sector at time t. The change in income compared to the initial period is given by:
where is the proportional change in average personal income in sector between and . We use information from COVID-19 rapid surveys conducted by GSS to estimate the proportional variation in income by sector.
We assume that household disposable income depends on the following factors: (i) the net income earned by each active household member, by economic sector, (ii) households’ internal and external remittances, (iii) public transfers (including those in place before COVID-19 broke out and those introduced after), and (iv) other factors depending on the availability of data. We assess the pandemic’s impact on welfare by estimating the amount of income that is earned in each sector and by each active household member. We draw on information from the GLSS about household members’ economic activity status and the economic sector in which they are active. Our results are reported by economic sector.
We estimate the food and beverage component of the CPI by forecasting CPI values based on past CPI values—an autoregressive approach that involves using a one-month lag in the CPI to obtain the optimistic scenario for prices and then adding 0.2 p. p to obtain the pessimistic scenario. Since the COVID-19 rapid surveys do not report actual wage changes, we instead used the observed change in sales, the probable and expected change in sales, and the share of labour (in terms of value added) from the 2015 Social Accounting Matrix. We used the survey self-reported pessimistic and optimistic expectations of business sales to estimate the predicted change in wages in our pessimistic and optimistic scenarios, which gives us:
where is the share of labour (value added),2 Sales represents reported sales, j indexes whether the scenario in question is pessimistic or optimistic, is the scenario, ESales is the business in question’s predicted change in sales.
We developed two scenarios for our simulations. The first scenario uses conservative estimates of the food price increases and income losses by sector from March to May 2020. The second scenario uses slightly higher prices, implying a more pessimistic scenario. The two scenarios are used in generating the results presented in the next section.
In each scenario, we calculate the level of welfare based on the change in the factors affecting welfare. We then estimate the level of poverty and inequality, and compare the welfare baseline with the simulated measure of welfare following the COVID-19 shocks and the simulated policy response.
In our first simulation, we adjust households’ spending on electricity and water to account for the payment of those utilities by the government.
In the second simulation, we randomly select self-employed individuals from the GLSS data who work in the creative arts industry, randomly assign them loans, and then calculate the new level of welfare. The National Board for Small Scale Industries (NBSSI) has indicated that over 15,228 beneficiaries received support, with food vendors, barbers, beauticians and dressmakers receiving up to a maximum of GHS2,000.3 We add the loan amount to the household welfare variable to determine the new level of household welfare.
In our third simulation, we simulate the waiving of taxes for health workers and the additional 50% income allowance they were granted. We compute each health worker’s gross income using the PAYE tax schedule. After obtaining their gross income, we calculate the difference between gross and reported income to get our estimate of the amount of taxes paid. The taxes plus 50% of their reported income are added to their income to determine the new level of welfare.
In the fourth simulation, we adjust the fees of all public university students considered absorbed by the government. We increase public university students’ spending on fees in the household’s spending basket by 50% to account for the subsidy.
After obtaining our new welfare measurements, we closely follow the approach by Araar (2006) to check the dominance in poverty and inequality. To estimate the difference in Lorenz curves,4 we compare the distributions of the old and new welfare measurements, that is, welfare prior to any policy simulation (Distribution 1) and welfare after a given policy has been simulated (Distribution 2). We would consider Distribution 2 to dominate Distribution 1 if Distribution 2 has a lower level of inequality than Distribution 1 everywhere on the welfare distribution (see, Araar, 2006):
where p is the percentile and Li(p) is the Lorenz curve for Distributions 1 and 2.
Foster, Greer and Thorbecke (FGT) poverty index curves require a monotonic transformation (PS(z)) of the following form to show dominance (Araar, 2006):
where c is a constant term. Distribution 2 dominates Distribution 1 in poverty for the order if
where is the FGT index for Distributions 1 and 2, and is the difference in the distribution of poverty between the two distributions.5
Female-headed households form 31.2% of the sample, which makes it possible to compare differences across households. We also analyse the sex of household members to complement the differences studied at the household level. As Boudet et al. (2018) note, one limitation of individual-level data is the limited differences in welfare that are observed at the individual level. Thus, we use data on household members for outcomes that involve more variation from person to person—when individual members make the choices. For example, electricity and water expenditures, unlike spending on mobile phone calls and other personal expenses, is made at the household level rather than the individual level, so a comparison by sex of the household head can provide insightful results. On the other hand, using information on individual household members allows us to show disaggregate effects on men, women and children.
5. Data and Measurement of Key Variables
We obtained the data required for our analysis from Round 7 of the GLSS (GLSS7), which was administered in 2017. The GLSS is a nationally representative sample of respondents living in Ghana at the time the survey is conducted.
We updated the expenditure values using January 2017 and March 2020 CPI values obtained from GSS.6 We updated sampling weights based on GSS population projections (total population, from 27.9 million in 2017 to 30.9 million in 2020).
We used averages computed from GSS COVID-19 rapid surveys: (a) COVID-19 Business Tracker Rapid Surveys and (b) COVID-19 Household and Job Tracker Rapid Surveys– to adjust our assumptions/values for job losses and changes in sales, profits, income and remittances. The information from the GSS COVID-19 rapid surveys is useful for choosing appropriate price increases for products and to project job losses suffered by households. The business tracker surveys were based on a sample of 4,311 (for Wave 1 – interviews conducted between May 26 and June 17, 2020) and 3,658 (for Wave 2 – interviews conducted between August 15 and September 10, 2020) household and non-household businesses. The household and job tracker surveys had two modules: (a) one on aggregate household effects and (b) one on changes within the household focusing on children. The sample size varied from one module to another, with Module A having the larger sample (3,265 respondents) than Module B (2,063 respondents). The samples are nationally representative and were collected in June 2020.
Our key variables are household’s estimate of food expenditures, non-food expenditures, total household expenditures, adult equivalent expenditures (welfare) and total household income. These variables allow us to estimate the effects of prices on household welfare and income using the methodology described above.
Finally, the GLSS7 provides limited information on household members’ income (only a small proportion of respondents—less than 21% of workers—reported their income). Given that national income conventions suggest an equivalence between income and expenditures, we predict income by sector based on a regression of total expenditures on a set of demographic and household variables (including age, region dummies, religion, sex, father’s education, father’s occupation, marital status, own education, an urban dummy, and own occupation) for single-member households. We use a Poisson pseudo-maximum likelihood estimator to ensure that our estimated expenditures are positive. The coefficients from the regression are then used to predict household income—which is used in calculating the relief granted to health workers and incorporating the change in income of economically active household members. Average total household expenditures were GHS 18,779.88, while the average estimated household income was GHS 19,422.05.
6. Results
6.1. Descriptive results
Changes in prices and income were key to the impact seen at the household level. Food price changes were forecasted based on the food component of the consumer price index (CPI) using monthly data from January 2012 to January 2021. There was a sharp increase in food and beverage prices between March and May 2020 as (year-on-year) inflation almost doubled from 8.4% to 15.1% (see Figure 2). Monthly inflation for food and beverages rose from 1.6% in March to 6.4% in Arezki et al. (2020) This steep increase in prices was one of the most important factors contributing to the significant worsening of welfare observed in our results below.7
Figure 3 shows the regional differences in inflation in March, April and May, which played a key role in the welfare losses suffered by households. As shown in Figure 4, food purchases form a significant component of the budget of most households across the expenditures deciles. We thus expect rising food prices to significantly decrease household welfare.
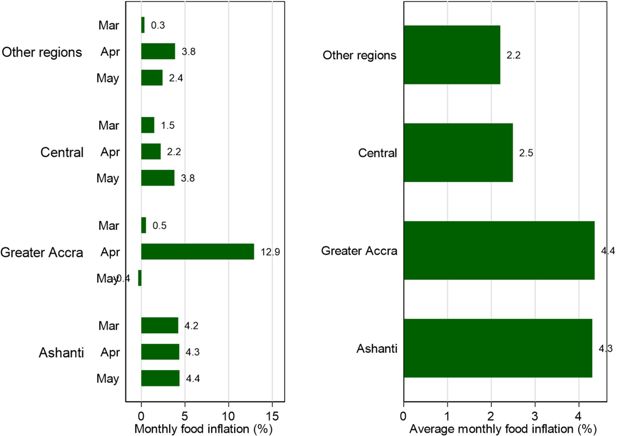
Regional inflation: Locked down versus non-locked down regions. Source: Authors’ calculation based on CPI data retrieved from www.statsghana.gov.gh
Apart from prices, household were also affected by income losses. More than 70 percent of rapid survey respondents indicated their remittances and income decreased during the first wave. However, fewer respondents (less than 7 percent) indicated their income decreased in the second wave (see Table A1 in Appendix A). The income losses are expected due to the decline in demand over the period (see Figure A1 in Appendix A). In our simulations, increasing prices and income losses together contributed approximately 90% to 95% of the impact on our welfare and poverty outcomes.
The baseline poverty rates differ by sex and age (see Figure 5). Males up to 24 years of age and those aged 40–44, 60–64, 68–72 and 80 or older experience relatively higher poverty than females of the same age bracket do. On the contrary, females aged 24–40, 44–60, 64–68 and 72–80 tend to experience relatively higher poverty than males of the same age bracket do. The distribution of poverty indicates significant vulnerability to poverty for both sexes and across the age brackets.
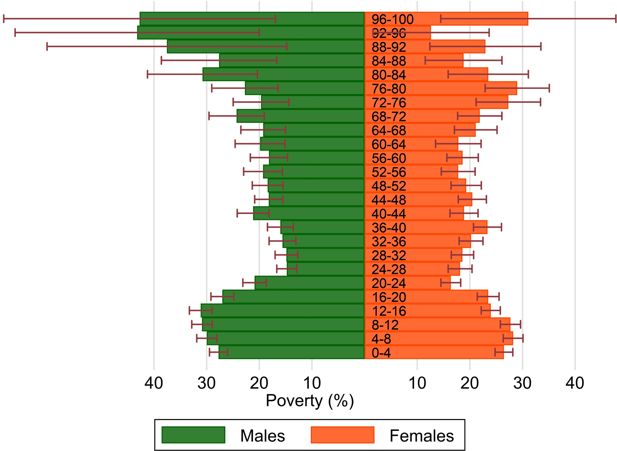
Distribution of poverty rates by age group. Notes: Apart from respondents aged 80 and over, of which there were 170 or fewer in each sex category, all other age categories had at least 183 male respondents and 320 female respondents. There were more than 1,124 respondents under age 40 in each sex category. Source: Authors’ own calculations based on GLSS7 survey by GSS.
6.2. COVID-19’s impact on poverty and inequality
We show four versions of the COVID-19’s impact on households, based on per adult equivalent consumption, poverty headcount, number of poor people and inequality for the first three months of COVID-19 (see Table 1). The results show that adjusting for food price variations across regions that were and were not under lockdown, leads to marginal differences in the results. For instance, consumption falls by 34.2% in the absence of regional price variations. However, when average food price variation for March to May 2020 is used, the reduction in consumption was 34.8%. Using the higher April price increases yields an increase of 36.3%. The three-month average food price variation value provides consistent impacts across the optimistic and pessimistic scenarios, and we adopt this approach for our analysis.
COVID-19’s impact on poverty and inequality – March to May 2020
Initial | Scenario 1 | Scenario 2 | |||
---|---|---|---|---|---|
Outcomes | Level | Level | Change (%) | Level | Change (%) |
No regional food CPI variation | |||||
Welfare (GHS) | 1,372.58 | 903.69 | -34.16%** | 830.09 | -39.52%* |
Number of poor (‘000s) | 7,255 | 10,033 | 2,778*** | 10,335 | 3,080*** |
Poverty headcount (% points) | 23.41% | 32.37% | 8.96%*** | 33.35% | 9.94%*** |
Gini | 0.43 | 0.88 | 0.44 | 1.01 | 0.58 |
Adjusted using average food CPI variation: March to May 2020 | |||||
Welfare (GHS) | 1,372.58 | 893.97 | -34.87%** | 819.23 | -40.31%** |
Number of poor (‘000s) | 7,255 | 10,094 | 2,839*** | 10,407 | 3,151*** |
Poverty headcount (% points) | 23.41% | 32.57% | 9.16%*** | 33.58% | 10.17%*** |
Gini | 0.43 | 0.88 | 0.45 | 1.02 | 0.59 |
Adjusted using food CPI variation: April 2020 | |||||
Welfare (GHS) | 1,372.58 | 874.06 | -36.32%** | 796.99 | -41.93%** |
Number of poor (‘000s) | 7,255 | 10,191 | 2,936*** | 10,503 | 3,247*** |
Poverty headcount (% points) | 23.41% | 32.88% | 9.47%*** | 33.89% | 10.48%*** |
Gini | 0.43 | 0.89 | 0.45 | 1.03 | 0.60 |
-
Notes: [1]- The poverty measurement is the headcount. [2]- * p < 0.10, ** p < 0.05, *** p < 0.01. [3]- Significance levels are reported only for the change statistic. [4]- Welfare is based on per adult equivalent consumption. [5] Poverty line = GHS 2326.596. Source: Authors’ own calculations based on GLSS7 survey by GSS.
The number of poor people increases by 2.8 million in the optimistic scenario and 3.1 million in the pessimistic scenario. Adjusting for food price variations across regions that were and were not under lockdown shows that the number of poor people increased by at least 2.8 million. Similarly, the poverty headcount and Gini index values increase in both scenarios—poverty, by at least 9 p. p, and inequality, by 0.45 points. We consider the results to be a lower bound for the pandemic’s impact due to the assumptions made and the fact that the effect could be larger if some of the channels through which the pandemic affects households were not captured. Increasing the period of analysis to December 2020 yields much lower impacts, and in some cases, a marginal reduction in poverty is observed. However, the reduction is not enough to offset the initial increase in poverty or reduction in welfare. We show further down a disaggregation of the results by various demographic factors and population (men, women and children).
6.3. Selected policy responses implemented by the government
The policies implemented (that is, loans for creatives, water and electricity subsidies, and tax reliefs for health professionals) marginally reduced poverty for both males and females (Table 2). The reliefs for health professionals benefitted women more than men. We observe a relatively larger reduction in poverty for women than men. If we compare the effects of all three policies, poverty decreases relatively more for men than women. Our simulation of the proposed 50% fee reduction for public university students shows the policy reduces poverty marginally and relatively more for men than women. One conclusion from our simulations is that the three policies implemented reduced poverty only marginally, less than 1 p. p. in all cases. This reduction is not adequate to offset the increase in poverty due to COVID-19.
Simulated effects of government policies for males and females
Total | Males | Female | |||||||
---|---|---|---|---|---|---|---|---|---|
Initial | Simulated | Change | Initial | Simulated | Change | Initial | Simulated | Change | |
50% fee reduction (March–December) | 23.41 | 23.41 | -0.005 | 24.13 | 24.13 | -0.007 | 22.73 | 22.73 | -0.003 |
Loans for creatives (March–December) | 23.41 | 23.41 | -0.004 | 24.13 | 24.13 | -0.006 | 22.73 | 22.73 | -0.003 |
Water/electricity subsidies (March–May) | 23.41 | 22.87 | -0.545 | 24.13 | 23.52 | -0.608 | 22.73 | 22.24 | -0.486 |
Water/electricity subsidies (March–December) | 23.41 | 23.11 | -0.302 | 24.13 | 23.78 | -0.354 | 22.73 | 22.48 | -0.253 |
Health reliefs (March–December) | 23.41 | 23.40 | -0.008 | 24.13 | 24.13 | -0.006 | 22.73 | 22.72 | -0.011 |
All three policies (March–December) | 23.41 | 23.10 | -0.315 | 23.41 | 23.10 | -0.315 | 22.73 | 22.46 | -0.267 |
-
Source: Authors’ own calculation based on GLSS7 survey by GSS.
In summarising the effects of the policies, we observe that the education subsidy reduces the number of poor people by 1,550, whereas the tax reliefs for health workers reduce the number of poor people by 2,479. The largest impact is provided by the water and electricity subsidies applied in the first three months, which reduce the number of poor people by 260,947. This shows the importance of the water and electricity subsidy (which was universal in nature) in cushioning some of the pandemic’s impact on households. However, the nine months of water and electricity subsidies reduce the number of poor people by 93,594. The creative loans, which had the lowest impact, reduce the number of poor people by 1,240.
We estimated the cost of the policy providing free water alone for nine months to amount to GHS 521.94 million, which is less than the amount the government reported (GHS 834 million). Our estimate excludes administrative costs and is based on an estimate of the current population. We therefore see our combined costs of the policy, of GHS 2.69 billion (or 0.73% of 2020 GDP), as a lower bound estimate. The loan to creatives is estimated to cost GHS 33.38 million. However, since repayment from beneficiaries is expected, this will not be a long-term cost for the government. The health workers’ reliefs was estimated to have cost GHS 918.18 million. The policies simulated must be scaled up and used in conjunction with additional policies to ensure a stronger reduction in poverty and inequality. Furthermore, the total costs of all three policies will increase the budget deficits by a minimum of GHS 3.7 billion, which is equivalent to 1% of 2020 GDP. The cost per poor person lifted out of poverty was lowest for the free water and electricity policy (GHS 13,362 to GHS 20,596) and highest for health sector reliefs (GHS 329,688). Even though the absolute costs of providing free water and electricity was the highest, it achieved a much lower cost per person after accounting for the reduction in poverty due to the policy.
In terms of equity, we find that the health reliefs provide more benefits to females than males, unlike the other two policies implemented, which benefit males relatively more than females (and the proposed public university fee subsidy which would have also benefited male students more than female students). A reason for this is that the health sector employs far more females than males and relatively more females were frontline health workers.
The results suggest the importance of policymakers better targeting poor groups and focusing on subsidising goods and services largely consumed by poor people. The fiscal costs will be lower and efficiency will be higher as more poor people will see an improvement in their welfare. For instance, rather than a universal electricity and water subsidy, it should be for the areas hardest hit by COVID-19 or for households that have a main income earner who was laid-off or have an income-earning member who died due to COVID-19 complications. Also, households with workers in the worst hit sectors, those with the highest layoffs, could be targeted and provided subsidised electricity and water (since some low-income households may consume more than the lifeline amount of electricity because they share their electricity meter with other households on their compound).
6.4. COVID-19s impact on adult male, adult female and child populations
Table 3 is based on the initial three months after the first few cases of COVID-19 were reported in Ghana (the first quarter). The results vary not only by population (men, women and children), but also by demographic characteristics such as sex of the household head, sector of work, household size, rural-urban area and whether the household was subject to the three-week lockdown that occurred in select regions.
COVID-19 and poverty headcount – men, women and children (March–May 2020)
Populations | Male | Female | Child | ||||||||||||
---|---|---|---|---|---|---|---|---|---|---|---|---|---|---|---|
Initial | Scenario 1 | Policies | Initial | Scenario 1 | Policies | Initial | Scenario 1 | Policies | |||||||
Groups: | Level | Level | Change | Level | Change | Level | Level | Change | Level | Change | Level | Level | Change | Level | Change |
Household size | |||||||||||||||
1-3 members | 5.47% | 15.43% | 9.95%*** | 5.33% | -0.14%*** | 6.95% | 14.67% | 7.73%*** | 6.62% | -0.32%** | 10.03% | 16.17% | 6.14%*** | 9.31% | -0.72% |
4-6 members | 18.76% | 28.78% | 10.02%*** | 18.05% | -0.70%** | 18.27% | 28.24% | 9.97%*** | 17.82% | -0.45%*** | 20.44% | 29.98% | 9.54%*** | 20.10% | -0.33%*** |
7-9 members | 35.66% | 45.16% | 9.51%*** | 35.31% | -0.34%* | 35.67% | 45.20% | 9.53%*** | 35.44% | -0.23%* | 37.02% | 45.69% | 8.67%*** | 36.83% | -0.19%* |
>9 members | 57.02% | 65.49% | 8.47%*** | 57.02% | 0.00% | 55.14% | 62.32% | 7.18%*** | 55.14% | 0.00% | 58.06% | 65.66% | 7.60%*** | 58.06% | 0.00% |
Sex of household head | |||||||||||||||
Male head | 21.11% | 30.79% | 9.68%*** | 20.74% | -0.37%** | 25.23% | 35.23% | 10.01%*** | 24.87% | -0.36%*** | 31.99% | 41.23% | 9.24%*** | 31.72% | -0.27%*** |
Female head | 18.69% | 29.06% | 10.37%*** | 18.07% | -0.63%** | 14.62% | 22.07% | 7.45%*** | 14.31% | -0.31%*** | 21.13% | 28.67% | 7.54%*** | 20.79% | -0.34%* |
Sector | |||||||||||||||
Agriculture forestry & fishing | 40.17% | 50.34% | 10.17%*** | 39.97% | -0.20%** | 42.80% | 52.69% | 9.89%*** | 42.57% | -0.23%** | 48.40% | 57.39% | 8.99%*** | 48.31% | -0.09%** |
Mining & quarrying | 12.58% | 2.20% | -10.37%* | 5.39% | -7.18% | 14.70% | 1.95% | -12.76%** | 10.60% | -4.10% | 19.33% | 4.42% | -14.91%** | 14.75% | -4.58% |
Manufacturing | 9.23% | 16.22% | 6.99%*** | 9.01% | -0.22% | 11.02% | 16.40% | 5.38%*** | 10.35% | -0.67%* | 15.99% | 21.74% | 5.75%*** | 15.12% | -0.87% |
Other manufacturing | 17.17% | 19.40% | 2.23% | 17.17% | 0.00% | 14.92% | 14.63% | -0.28% | 14.92% | 0.00% | 25.39% | 25.75% | 0.36% | 25.39% | 0.00% |
Construction | 7.29% | 11.86% | 4.57%*** | 7.24% | -0.05% | 10.39% | 15.88% | 5.49%*** | 10.33% | -0.06% | 13.37% | 18.02% | 4.65%*** | 13.29% | -0.07% |
Utility & gas | 4.39% | 6.29% | 1.90% | 4.39% | 0.00% | 5.56% | 8.91% | 3.35% | 5.56% | 0.00% | 0.00% | 1.33% | 1.33% | 0.00% | 0.00% |
Trade & repairs | 6.12% | 14.65% | 8.53%*** | 5.85% | -0.26%* | 7.03% | 16.18% | 9.16%*** | 6.93% | -0.10%* | 9.13% | 17.53% | 8.40%*** | 9.06% | -0.07% |
Restaurant & hotels | 9.32% | 18.14% | 8.82%*** | 9.19% | -0.13% | 7.13% | 15.95% | 8.82%*** | 6.95% | -0.19% | 11.08% | 15.87% | 4.78%** | 10.28% | -0.81% |
Education | 5.62% | 52.06% | 46.43%*** | 4.74% | -0.88% | 4.99% | 46.71% | 41.71%*** | 4.42% | -0.57% | 8.27% | 50.10% | 41.83%*** | 8.00% | -0.27% |
Entertainment & recreation | 9.89% | 12.90% | 3.01%** | 8.86% | -1.03% | 11.00% | 16.36% | 5.36%* | 10.34% | -0.66% | 24.07% | 27.33% | 3.26% | 20.50% | -3.57% |
Other services | 6.89% | 17.34% | 10.45%*** | 6.53% | -0.36%* | 7.03% | 14.97% | 7.94%*** | 6.63% | -0.40%* | 8.56% | 17.36% | 8.80%*** | 8.28% | -0.28% |
Area | |||||||||||||||
Rural | 35.82% | 45.71% | 9.89%*** | 35.49% | -0.34%*** | 37.51% | 46.55% | 9.05%*** | 37.06% | -0.44%*** | 44.69% | 53.05% | 8.36%*** | 44.42% | -0.27%*** |
Urban | 6.89% | 16.57% | 9.68%*** | 6.42% | -0.47%* | 6.83% | 15.82% | 8.98%*** | 6.59% | -0.25%** | 9.91% | 19.02% | 9.11%*** | 9.60% | -0.31%* |
Lockdown | |||||||||||||||
Not locked down | 29.68% | 41.02% | 11.35%*** | 29.12% | -0.55%** | 29.83% | 40.43% | 10.60%*** | 29.39% | -0.44%*** | 37.78% | 47.74% | 9.96%*** | 37.39% | -0.39%*** |
Locked down | 5.86% | 13.02% | 7.16%*** | 5.70% | -0.16%* | 6.51% | 12.87% | 6.36%*** | 6.34% | -0.17% | 10.21% | 16.42% | 6.20%*** | 10.11% | -0.11% |
Population | 20.76% | 30.54% | 9.78%*** | 20.35% | -0.40%*** | 21.09% | 30.10% | 9.01%*** | 20.75% | -0.34%*** | 28.58% | 37.29% | 8.71%*** | 28.29% | -0.29%*** |
-
Notes: [1]- The poverty measurement is the headcount. [2]- * p < 0.10, ** p < 0.05, *** p < 0.01. [3]- Significance levels are reported only for the change statistic. [4]- In p. p. [5]- Excludes the university fee subsidy. [6] Poverty line = GHS 2326.596. Source: Authors’ own calculations based on GLSS7 survey by GSS.
The pandemic’s impact was more severe for men living in areas that were not locked down for three weeks (+11.4 p. p.) than men living in locked down areas (7.2 p. p.). Similarly, women (10.6 vs 6.4 p. p.) and children (9.96 vs 6.2 p. p.) living in non-locked-down regions experienced a relatively higher increase in poverty compared to those in locked down areas. Note that poverty was already higher in non-locked down areas (29.7%) than the locked down areas (5.9%) prior to the pandemic.
Despite the lower impact on men, women and children in the locked down areas, the incidence of poverty in the locked down areas was among the lowest in the country and remains lower than in the other regions even after the COVID-19 shock. We provide a possible explanation for our results; however, a caveat is that microsimulation results tend to rely heavily on the assumptions made in the analysis. As a result, explanations have to take into account the assumptions made and the mechanisms through which the effects occur. One possible explanation for the lower incidence in the locked down areas could be because men, women and children in the non-locked-down regions found themselves relatively closer to the poverty line and therefore more vulnerable to poverty and more likely to become poor due to the COVID-19 shock. Another possible reason could be that the reduction in transfers from migrant families living in the regional capitals of Greater Accra and the Ashanti region allowed some households to rely on their savings and therefore fewer to reach consumption levels closer to the poverty line. Thus, recipients of the transfers in the other regions lost a source of income that allowed their welfare to rise or stay above the poverty line.
Men, women and children in households working in the mining and quarrying sector were not severely affected by the pandemic. In fact, we observe a reduction in poverty in that sector. Most of the mining concerns and quarrying sites are located outside the locked down areas (with the exception of mines on the outskirts of Greater Kumasi) and were thus not seriously affected by the lockdown measures. The same cannot be said for all the other sectors, which saw an increase in poverty among men, women and children. Those in the education sector were the most heavily affected, with men experiencing a relatively higher increase in poverty (46.4 p. p) compared to women (41.7 p. p.) and children (41.9 p. p.). The education sector was closed for almost a year except for national exam candidates being allowed to return to school later in the year to write their final exit exams. More importantly, for private education providers, the lack of teaching meant a loss of revenue and an inability to pay their teachers, unlike government-assisted schools whose teachers were paid by the government. Private universities found themselves in a similar situation, as they faced challenges resuming academic work online.
Other sectors that were adversely affected include the restaurant and hotel, entertainment and recreation, other services, manufacturing, other manufacturing, agriculture, utility and gas, trade, and repairs and construction sectors—which had headcount index values between 0.4 and 10.5 p. p. Men, women and children in households operating in those sectors saw an increase of at least 4 p. p. in poverty headcount. The utility and gas sector saw the lowest increase in poverty headcount for men and women, while children living in households with members operating in the “other manufacturing” sector saw the lowest increase in poverty headcount (0.4 p. p.). Women in the “other manufacturing” sector experienced a marginal decline in headcount index (-0.3 p. p.). It was not expected that those in the agriculture, forestry and fishing sectors would see the second largest increase in headcount poverty. However, market imperfections and the rising costs of transporting food products to and within the locked down areas might explain why the agriculture, forestry and fishing sector was significantly affected by the pandemic.
Men, women and children in mid-sized households (4-6 members) saw the largest increase in headcount index. Men and women in small households (1-3 members) were not the least affected by the pandemic; however, children in those households experienced the lowest increase in poverty headcount. While men and women living in rural areas saw a marginally higher increase in poverty than those in urban areas, children in urban areas saw a relatively higher increase in headcount index than those in rural areas. Across all three populations, rural poverty levels were more than five times higher than the corresponding urban poverty values (with children in rural areas having a headcount approximately 4.5 times that of children in urban areas).
We can also observe that the policies implemented by the government were heterogeneous across the populations and demographic characteristics. The larger households did not receive as many benefits as the smaller ones. Children in small households received as much as two times the benefits children in medium-sized households did.
Men in female-headed households, women in male-headed households and children in male-headed households saw relatively larger increases in headcount index. The policies yielded relatively larger reductions for the most affected sex across the household head category even though the policies’ effects are less than one percent. On the other hand, the policies reduced the headcount index for children in female-headed households relatively more than those in male-headed households despite the larger increase in poverty among male-headed households.
In analysing the pandemic’s impact over the period from March to December 2020 (see Table 4), we observe qualitatively similar results. However, the magnitude of the effects becomes smaller. For instance, men, women and children in locked down areas experience a relatively smaller increase in poverty (1.4 p. p., 2.9 p. p. and 3.1 p. p., respectively) compared to those in non-locked down area (3 p. p., 1.2 p.p and 1.2 p. p, respectively). The lower values can be attributed to the decrease in prices observed after May. Falling prices were more prevalent and prices fell faster in the locked down areas than the non-lockdown areas, which saw prices fall at a slower pace due to price stickiness and market imperfections. Also, as business activities picked up again in the locked down areas, they were more likely to be relieved of the pressure they initially experienced due to decreased income and remittances and rising prices.
COVID-19 and poverty headcount – men, women and children (March–December 2020)
Populations | Male | Female | Child | ||||||||||||
---|---|---|---|---|---|---|---|---|---|---|---|---|---|---|---|
Initial | Scenario 1 | Policies | Initial | Scenario 1 | Policies | Initial | Scenario 1 | Policies | |||||||
Groups: | Level | Level | Change | Level | Change | Level | Level | Change | Level | Change | Level | Level | Change | Level | Change |
Household size | |||||||||||||||
1-3 members | 5.47% | 7.31% | 1.84%*** | 5.33% | -0.14%*** | 6.95% | 8.52% | 1.58%*** | 6.62% | -0.32%** | 10.03% | 11.48% | 1.45%*** | 9.31% | -0.72% |
4-6 members | 18.76% | 21.26% | 2.50%*** | 18.05% | -0.70%** | 18.27% | 20.80% | 2.53%*** | 17.82% | -0.45%*** | 20.44% | 23.20% | 2.76%*** | 20.10% | -0.33%*** |
7-9 members | 35.66% | 38.88% | 3.23%*** | 35.31% | -0.34%* | 35.67% | 38.72% | 3.05%*** | 35.44% | -0.23%* | 37.02% | 39.82% | 2.80%*** | 36.83% | -0.19%* |
>9 members | 57.02% | 59.11% | 2.09%** | 57.02% | 0.00% | 55.14% | 56.30% | 1.16% | 55.14% | 0.00% | 58.06% | 59.30% | 1.24% | 58.06% | 0.00% |
Sex of household head | |||||||||||||||
Male head | 21.11% | 23.37% | 2.27%*** | 20.74% | -0.37%** | 25.23% | 27.79% | 2.56%*** | 24.87% | -0.36%*** | 31.99% | 34.37% | 2.38%*** | 31.72% | -0.27%*** |
Female head | 18.69% | 21.81% | 3.12%*** | 18.07% | -0.63%** | 14.62% | 16.33% | 1.71%*** | 14.31% | -0.31%*** | 21.13% | 23.73% | 2.59%*** | 20.79% | -0.34%* |
Sector | |||||||||||||||
Agriculture forestry & fishing | 40.17% | 43.49% | 3.31%*** | 39.97% | -0.20%** | 42.80% | 46.08% | 3.28%*** | 42.57% | -0.23%** | 48.40% | 51.50% | 3.11%*** | 48.31% | -0.09%** |
Mining & quarrying | 12.58% | 3.28% | -9.29% | 5.39% | -7.18% | 14.70% | 4.04% | -10.66%** | 10.60% | -4.10% | 19.33% | 8.25% | -11.08%* | 14.75% | -4.58% |
Manufacturing | 9.23% | 11.33% | 2.11%*** | 9.01% | -0.22% | 11.02% | 12.30% | 1.28%*** | 10.35% | -0.67%* | 15.99% | 17.63% | 1.64%*** | 15.12% | -0.87% |
Other manufacturing | 17.17% | 16.53% | -0.65% | 17.17% | 0.00% | 14.92% | 13.81% | -1.10% | 14.92% | 0.00% | 25.39% | 24.65% | -0.74% | 25.39% | 0.00% |
Construction | 7.29% | 8.74% | 1.45%** | 7.24% | -0.05% | 10.39% | 11.54% | 1.15%** | 10.33% | -0.06% | 13.37% | 14.69% | 1.32%* | 13.29% | -0.07% |
Utility & gas | 4.39% | 4.39% | 0.00% | 4.39% | 0.00% | 5.56% | 5.56% | 0.00% | 5.56% | 0.00% | 0.00% | 0.00% | 0.00% | 0.00% | 0.00% |
Trade & repairs | 6.12% | 7.93% | 1.82%*** | 5.85% | -0.26%* | 7.03% | 9.23% | 2.20%*** | 6.93% | -0.10%* | 9.13% | 11.61% | 2.48%*** | 9.06% | -0.07% |
Restaurant & hotels | 9.32% | 11.68% | 2.36% | 9.19% | -0.13% | 7.13% | 9.31% | 2.17%** | 6.95% | -0.19% | 11.08% | 12.12% | 1.04% | 10.28% | -0.81% |
Education | 5.62% | 12.86% | 7.24%*** | 4.74% | -0.88% | 4.99% | 11.61% | 6.61%*** | 4.42% | -0.57% | 8.27% | 16.95% | 8.68%*** | 8.00% | -0.27% |
Entertainment & recreation | 9.89% | 10.61% | 0.72% | 8.86% | -1.03% | 11.00% | 11.91% | 0.91% | 10.34% | -0.66% | 24.07% | 24.07% | 0.00% | 20.50% | -3.57% |
Other services | 6.89% | 8.89% | 2.00%*** | 6.53% | -0.36%* | 7.03% | 8.39% | 1.35%*** | 6.63% | -0.40%* | 8.56% | 10.34% | 1.78%*** | 8.28% | -0.28% |
Area | |||||||||||||||
Rural | 35.82% | 38.92% | 3.10%*** | 35.49% | -0.34%*** | 37.51% | 40.31% | 2.81%*** | 37.06% | -0.44%*** | 44.69% | 47.60% | 2.91%*** | 44.42% | -0.27%*** |
Urban | 6.89% | 8.62% | 1.74%*** | 6.42% | -0.47%* | 6.83% | 8.57% | 1.73%*** | 6.59% | -0.25%** | 9.91% | 11.81% | 1.90%*** | 9.60% | -0.31%* |
Lockdown | |||||||||||||||
Not locked down | 29.68% | 32.68% | 3.01%*** | 29.12% | -0.55%** | 29.83% | 32.69% | 2.86%*** | 29.39% | -0.44%*** | 37.78% | 40.83% | 3.05%*** | 37.39% | -0.39%*** |
Locked down | 5.86% | 7.22% | 1.36%*** | 5.70% | -0.16%* | 6.51% | 7.70% | 1.19%*** | 6.34% | -0.17% | 10.21% | 11.44% | 1.23%*** | 10.11% | -0.11% |
Population | 20.76% | 23.15% | 2.39%*** | 20.35% | -0.40%*** | 21.09% | 23.32% | 2.23%*** | 20.75% | -0.34%*** | 28.58% | 31.03% | 2.44%*** | 28.29% | -0.29%*** |
-
Notes: [1]- The poverty measure is the headcount. [2]- * p < 0.10, ** p < 0.05, *** p < 0.01. [3]- Significance levels are reported only for the change statistic. [4]- In p. p. [5]- Excludes the university fee subsidy. [6] Poverty line = GHS 2326.596. Source: Authors’ own calculations based on GLSS7 survey by GSS.
Over the longer period, children in urban households saw an increase in headcount that was relatively lower than those in rural households. The impact on education became relatively lower, as some private universities and primary and secondary schools resumed classes online, thereby providing the owners of the schools with revenue to pay their teachers for teaching online. The “other manufacturing” sector saw a marginal decrease in headcount for men, women and children, while the utility and gas sector did not show any significant changes. The increase in headcount became relatively smaller for small households (1-3 members) than medium-sized households (4-6 members) and larger households (7 and above). Women and children in large households saw a much lower increase in poverty.
Given the increases in poverty headcount, welfare would be down for men, women and children (see Table 5). If we use the per adult equivalent consumption measure, we find this to be the case. We find the smallest reduction in welfare among households with 7-9 and 9 or more members for men, women and children. In Table 5, households with 4-6 members saw the largest impact for men (-66.6%) and women (-77.3%), whereas small households saw the largest impact for children (-15.5%).
COVID-19 and welfare (per adult equivalent consumption) – men, women and children
Populations | Male | Female | Child | ||||||||||||
---|---|---|---|---|---|---|---|---|---|---|---|---|---|---|---|
Initial | Scenario 1 | Policies | Initial | Scenario 1 | Policies | Initial | Scenario 1 | Policies | |||||||
Groups: | Level | Level | Change | Level | Change | Level | Level | Change | Level | Change | Level | Level | Change | Level | Change |
Household size | |||||||||||||||
1-3 members | 2232.37 | 1739.06 | -22.10%*** | 2268.54 | 1.62%*** | 2139.02 | 1549.34 | -27.57%*** | 2189.39 | 2.35%*** | 1694.12 | 1431.86 | -15.48%*** | 1715.83 | 1.28%*** |
4-6 members | 1345.45 | 449.53 | -66.59% | 1360.09 | 1.09%*** | 1383.73 | 313.74 | -77.33% | 1399.7 | 1.15%*** | 1285.68 | 1105.85 | -13.99%*** | 1298.3 | 0.98%*** |
7-9 members | 977.25 | 831.02 | -14.96%*** | 983.2 | 0.61%*** | 954.84 | 813.53 | -14.80%*** | 962.65 | 0.82%*** | 905.55 | 780.96 | -13.76%*** | 911.53 | 0.66%*** |
>9 members | 655.36 | 557.23 | -14.97%*** | 658.04 | 0.41%*** | 663.3 | 570.98 | -13.92%*** | 665.99 | 0.41%*** | 611.28 | 526.65 | -13.85%*** | 613.82 | 0.41%*** |
Sex of household head | |||||||||||||||
Male head | 1531.49 | 902.73 | -41.06%* | 1550.27 | 1.23%*** | 1310.77 | 453.29 | -65.42% | 1325.59 | 1.13%*** | 1087.08 | 928.38 | -14.60%*** | 1095.8 | 0.80%*** |
Female head | 1326.22 | 1119.18 | -15.61%*** | 1345.71 | 1.47%*** | 1712.89 | 1293.6 | -24.48%*** | 1749.7 | 2.15%*** | 1256.97 | 1089.44 | -13.33%*** | 1271.35 | 1.14%*** |
Sector | |||||||||||||||
Agriculture forestry & fishing | 888.38 | 747.24 | -15.89%*** | 894.01 | 0.63%*** | 835.31 | 679.28 | -18.68%*** | 840.38 | 0.61%*** | 718.62 | 625.57 | -12.95%*** | 722.26 | 0.51%*** |
Mining & quarrying | 1775.99 | 1996 | 12.39%*** | 1802.31 | 1.48%*** | 1608.55 | 1756.38 | 9.19%*** | 1643.18 | 2.15% | 1591.3 | 1704.4 | 7.11%*** | 1639.63 | 3.04% |
Manufacturing | 1654.79 | 1419.06 | -14.25%*** | 1669.93 | 0.92%*** | 1667.47 | 1454.49 | -12.77%*** | 1681.6 | 0.85%*** | 1302.72 | 1151.86 | -11.58%*** | 1311.5 | 0.67%*** |
Other manufacturing | 1478.85 | 1331.85 | -9.94%*** | 1491.39 | 0.85%*** | 1423.91 | 1235.62 | -13.22%*** | 1434.97 | 0.78%*** | 1165.11 | 1016.8 | -12.73%*** | 1177.28 | 1.04%*** |
Construction | 1717.57 | 1525.4 | -11.19%*** | 1730.96 | 0.78%*** | 1529.16 | 1349.71 | -11.74%*** | 1539.53 | 0.68%*** | 1504.25 | 1348.89 | -10.33%*** | 1513.07 | 0.59%*** |
Utility & gas | 2476.22 | 2376.49 | -4.03%** | 2497.97 | 0.88%*** | 2316.47 | 2143.64 | -7.46%*** | 2330.62 | 0.61%*** | 2007.7 | 1871.2 | -6.80%*** | 2021.35 | 0.68%*** |
Trade & repairs | 1933.54 | 1576.61 | -18.46%*** | 1950.08 | 0.86%*** | 1794.99 | 1501.84 | -16.33%*** | 1810.3 | 0.85%*** | 1465.8 | 1257.72 | -14.20%*** | 1476.71 | 0.74%*** |
Restaurant & hotels | 1492.37 | 1264.79 | -15.25%*** | 1506.3 | 0.93%*** | 1736.59 | 1512.57 | -12.90%*** | 1752.86 | 0.94%*** | 1456.69 | 1310.14 | -10.06%*** | 1468.7 | 0.82%*** |
Education | 1976.82 | 714.9 | -63.84%*** | 1991.06 | 0.72%*** | 1995.56 | 997.76 | -50.00%*** | 2010.44 | 0.75%*** | 1575.84 | 883.61 | -43.93%*** | 1584.66 | 0.56%*** |
Entertainment & recreation | 2334.86 | 1952.84 | -16.36%*** | 2396.13 | 2.62%*** | 2139.94 | 1857.97 | -13.18%*** | 2182.72 | 2.00%*** | 1765.63 | 1599.03 | -9.44%*** | 1801.31 | 2.02%*** |
Other services | 2375.4 | 1816.72 | -23.52%*** | 2461.45 | 3.62%*** | 2378.23 | 1899.19 | -20.14%*** | 2526.26 | 6.22%*** | 1872.05 | 1540.17 | -17.73%*** | 1918.93 | 2.50%*** |
Area | |||||||||||||||
Rural | 1030.97 | 244.55 | -76.28% | 1039.93 | 0.87%*** | 980.96 | -132.58 | -113.52% | 995.8 | 1.51%*** | 787.82 | 680.36 | -13.64%*** | 792.61 | 0.61%*** |
Urban | 1935.86 | 1568.73 | -18.96%*** | 1963.88 | 1.45%*** | 1890.11 | 1574.22 | -16.71%*** | 1920.93 | 1.63%*** | 1549.15 | 1325.06 | -14.47%*** | 1566.26 | 1.10%*** |
Lockdown | |||||||||||||||
Not locked down | 1152.34 | 467.59 | -59.42% | 1166.47 | 1.23%*** | 1118.48 | 215.21 | -80.76% | 1136.93 | 1.65%*** | 890.43 | 763.37 | -14.27%*** | 898.1 | 0.86%*** |
Locked down | 2086.16 | 1712.75 | -17.90%*** | 2112.98 | 1.29%*** | 2049.75 | 1724.49 | -15.87%*** | 2081.4 | 1.54%*** | 1639.71 | 1409.51 | -14.04%*** | 1655.87 | 0.99%*** |
Population | 1502 | 933.83 | -37.83%** | 1520.88 | 1.26%*** | 1467.6 | 781.02 | -46.78%* | 1491 | 1.59%*** | 1140.38 | 978.91 | -14.16%*** | 1150.88 | 0.92%*** |
-
Notes: [1]- The poverty measure is the headcount. [2]- * p < 0.10, ** p < 0.05, *** p < 0.01. [3]- Significance levels are reported only for the change statistic. [4]- In p. p. [5]- Excludes the university fee subsidy. [6] Poverty line = GHS 2326.596. Source: Authors’ own calculations based on GLSS7 survey by GSS.
Inequality increased for men (0.5 p. p.) and children (0.4 p. p.). Although the estimate for women is positive, it is not well-determined since it is statistically insignificant and quite high, at 0.9 p. p.
One caveat of our results is that when those who are vulnerable are already in poverty, we are unlikely to see a considerable increase in the poverty headcount. On the contrary, in the categories that have fewer people in poverty and those above the poverty line are considerably above it, we are unlikely to see a significant increase in the headcount index. When we observe significant increases in the index, it suggests that quite a number of people are quite close to the poverty line and any income or price shocks they face in the market push them into poverty.
Figure 6 summarizes the pandemic’s effects on poverty and welfare. As shown in the charts, the effects vary for men, women and children. The decrease in welfare is relatively larger for women (46.8%) than men (37.8%) and children (14.2%). Although the three polices implemented marginally increase welfare, they provide a relatively larger increase for women than men and children. On the contrary, the increase in poverty in the first three months is larger for men (9.8 p.p.) than women (9 p p.) and children (8.7 p. p.). However, if we consider the pandemic’s impact on poverty from March until December, the increase in poverty is marginally higher for children (2.44 p. p.) than men (2.39 p. p.) and women (2.2 p. p.).
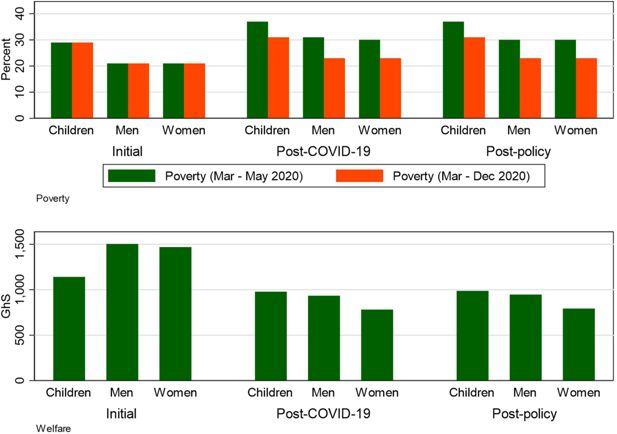
Summary of the impact of COVID-19 and selected policy responses. Notes: [1] “Post-policy” excludes the university fee subsidy. [2]- The bars represent the level of the welfare outcome, with “Initial” being the baseline outcome, “Post-COVID-19” being the level after COVID-19, and “Post-policy” being the level after COVID-19 and the implementation of the policies selected. [3] Poverty line = GHS 2326.596. Source: Authors’ own calculations based on GLSS7 survey by GSS.
7. Conclusions and policy implications
The COVID-19 pandemic led to job losses, more people falling into poverty, students missing key periods in their educational development journey and exposed the limited health infrastructure available in most African countries.
Our simulation of the first three and nine months after the first case of COVID-19 was confirmed in Ghana shows the pandemic resulted in an increase in poverty, a decrease in household welfare and an increase in inequality. Its effect varies by sector of work, sex and area, and depending on whether the region was locked down. The hardest-hit sector in terms of welfare loss and poverty increase was the education sector. The remaining sectors, apart from the mining and quarrying sector, and to a lesser extent the “other manufacturing” sector, also saw higher poverty and lower welfare for men, women and children. We find that in the short term after the pandemic, those in regions that were not locked down and in rural areas experienced a relatively higher poverty incidence. If we extend our simulation to the end of the year (December 2020), the results are similar in terms of sectoral impacts and across groups (including men, women and children), with a few exceptions.
We simulated the following policies: (a) the provision of free electricity to lifeline consumers and free water to all households; (b) the provision of loans to workers in the creative arts industry; and (c) the provision of tax and other reliefs to health workers. We also added an additional policy—the absorption of 50% of public university fees—that was considered but ultimately not implemented by the government. All three implemented policies had some effect in marginally reducing poverty, with the highest reduction being 0.55 p. p. attributable to the provision of three months of free water and a 50% tariff reduction on electricity. The other policies reduced poverty much less due to their limited household coverage. Additional policies that directly target the poor, such as free electricity for lifeline consumers, are important to mitigate the increase in poverty due to COVID-19. Our estimates of costs indicate that the loans to creatives was the cheapest policy (GHS 33.38 million) and the free water and electricity policy was the most expensive (GHS 2.69 billion). Free water alone cost GHS 521.9 million. The relief to health workers cost GHS 918.2 million. However, when we account for the reduction in poverty, the free water and electricity policy generates the lowest costs per person lifted out of poverty of GHS 13,362 to GHS 20,596 while it is highest for the reliefs for health workers (GHS 329,688). Our costs are lower bound estimates and exclude the administrative costs to administer the policies.
Our welfare results are qualitatively similar to those of Aduhene and Osei-Assibey (2021), Bukari et al. (2021) and Schotte et al. (2021). Like these studies, we find poverty and inequality rise and welfare falls. While we report a 34.2% to 36.3% decline in welfare, Bukari et al. (2021) arrived at an 18% decline in welfare.
Our assumptions about prices, income, remittances, public transfers and other factors can affect our simulation of the pandemic’s impact on our welfare outcomes. If the factors are underestimated, then the changes in the factors would lead to underestimating the pandemic’s impact.
Footnotes
1.
2.
Labour value-added in the SAM includes wage earnings, farm and non-farm enterprise revenues.
3.
4.
We calculate the Gini values using conventional methods. Since we use per adult equivalent expenditures as our measure of welfare, it is implicitly assumed there is no intra-household inequality.
5.
We use an absolute poverty line based on the GSS’s national poverty line. We adjust the poverty line to 2020 prices (National poverty line: GHS 1760.86; adjusted poverty line: GHS 2326.596 (upper)).
6.
Since the basket and base year of the CPI series were revised, we use January 2017 and July 2019, which are based on the old series, with August 2019 and March 2020, which are based on the new series, to obtain the appropriate factor by which to increase the expenditures and poverty line values. We also use estimated population growth figures from GSS to update the sample weights.
7.
The large price variation could underestimate the welfare effect. Araar and Verme (2019) discussion that, the Cobb-Douglas model tends to provide reasonable estimates in the presence of high price increases and is comparatively better than Hicks approximations. It, however, may underestimate the welfare effect when compared to more complicated and sophisticated demand systems.
Appendices
Appendix A
Self-reported changes in income by source
Nature of change and change in % | ||||||
---|---|---|---|---|---|---|
Increased | No change | Decreased | ||||
Period | Wave 1 | Wave 2 | Wave 1 | Wave 2 | Wave 1 | Wave 2 |
Wages | 3.2 | 44.9 | 41.8 | 49.6 | 55 | 5.5 |
Foreign remittances | 2.9 | 65.4 | 17.2 | 28.3 | 79.9 | 6.3 |
Domestic remittances | 2.3 | 58.9 | 27 | 37.2 | 70.7 | 4.9 |
Change in income | 3.1 | 65.9 | 19.4 | 27.8 | 77.4 | 6.3 |
-
Notes: Sample weights are applied. The reference time is March 16, 2020, for both waves.
-
Source: Authors’ own calculations based on GSS Covid-19 rapid surveys
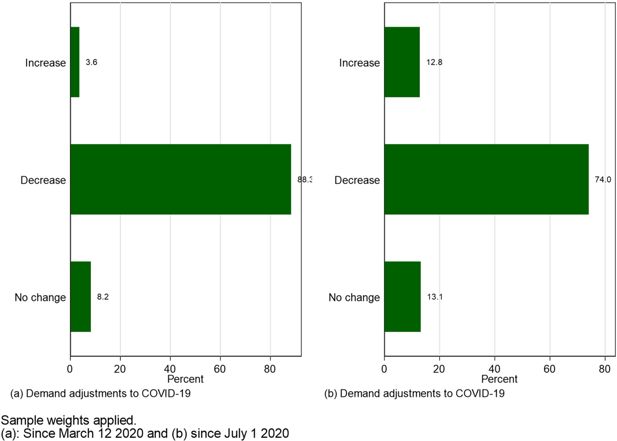
Demand adjustments due to COVID-19. Source: Authors’ own calculations based on GSS Covid-19 rapid surveys
Appendix B
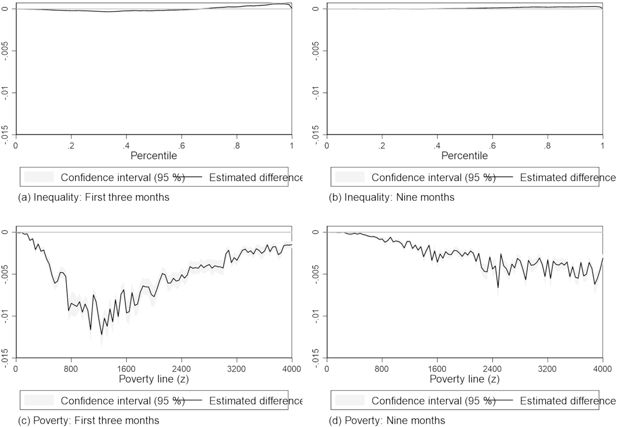
Free water and free electricity for lifeline customers. Source: Authors’ own calculations based on GLSS7 survey by GSS.
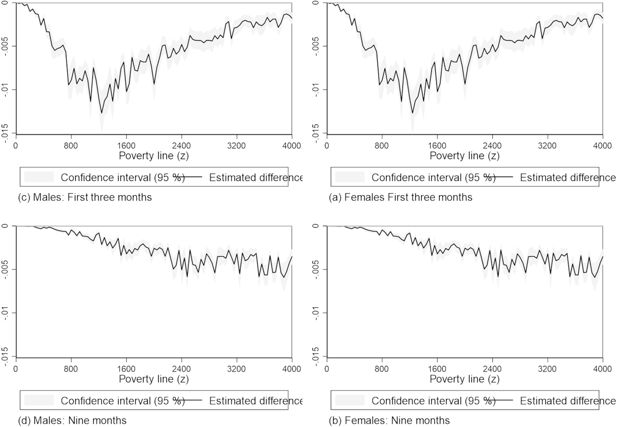
Free water and free electricity for lifeline customers by sex. Note: Comparison made based on the sex of the household head. Source: GLSS7
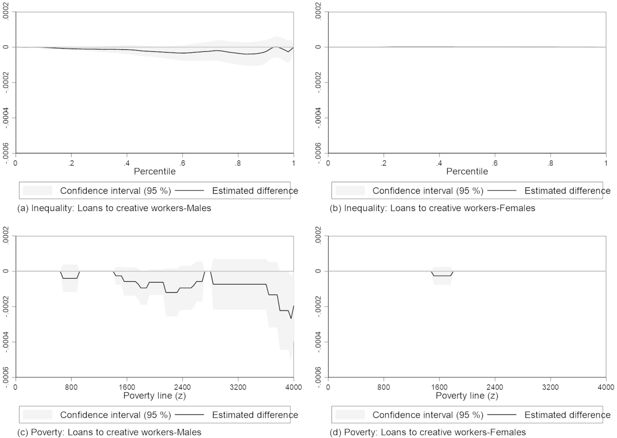
Simulation of loans to creatives. Source: Authors’ own calculations based on GLSS7 survey by GSS.
Appendix C
List of assumptions, adjustments and potential implications
Item | Assumptions | Adjustments | Average changes | Implications |
---|---|---|---|---|
Labour income | Equivalence between income and expenditures. | 1. Predicted via a regression to adjust the values reported in the survey for potential measurement errors and assigning individual income to household members. 2. Hot deck approach adopted to correct for missing values. 3. Adjusted upwards from 2018 to 2020 using CPI values for the year of the survey. 4. Changes in income computed using mean values from GSS rapid surveys for sales, and self-reported optimistic and pessimistic probabilities of sales changes. It provides us with more realistic assumptions about income changes due to COVID-19. | 1. Inflation adjustment: 32.13% 2. Pessimistic probability of expected change in sales in %: Agri. (21.5) Mining (20.5) Manufacturing (29.3) Other manufacturing (30.0) Construction (29.2) Utility (62.2) Trade (23.6) Restaurant and hotel (44.4) Education (26.3) Entertainment (50.0) Other services (28.9) 3. Optimistic probability of expected change in sales in %: Agri. (33.3) Mining (11.5) Manufacturing (22.7) Other manufacturing (40.0) Construction (37.2) Utility (60.0) Trade (46.3) Restaurant and hotel (33.8) Education (47.8) Entertainment (70.0) Other services (41.6) | 1. Likely to be understated or overstated based on the adjustments made. 2. Some sectors may not have adequate observations to enable hot deck imputation to provide reasonable values for missing observations. |
Water subsidy | Households with reported water bill payments are assumed to obtain their water from Ghana water company. | 1. Updated to current prices prior to the pandemic. 2. Hot deck imputation used for missing values. | Inflation adjustment: 32.13% | Not all households use Ghana water company. Some households miss out on the subsidy. Implications: 1. Fewer rural households compared to urban households will benefit. 2. It does not account for periods households do not receive water. |
Electricity subsidy | Positive estimates of an electricity bill are taken to be users of the national grid. | 1. Updated to current prices prior to the pandemic. 2. Hot deck imputation used for missing observations. | Inflation adjustment: 32.13% | Not all households use electricity from the grid. Implications: 1. Fewer rural households compared to urban households will benefit. 2. It does not account for power rationing actual electricity consumed. |
Loans to creatives | 1. Randomly sampled. 2. Same amount applied to all borrowers. | Males: 16,280 Females: 411 Overall: 0.054% of total population | 1. Under-reporting likely to underestimate the impact. 2. Does not take into account that loan recipients choose the amount to borrow. | |
Health reliefs | 1. We assume that the income values used in the analysis are a true reflection of actual income paid to health workers. 2. Equivalence of income and expenditures. | 1. Income values predicted via regression. 2. Taxes computed based on predicted income values. | Inflation adjustment: 32.13% | Potential understatement or overstatement of benefits, depending on whether aggregated categories of health professionals are excluded from the benefits. |
Prices | 1. Price increases follow the food CPI component. 2. Regional differences are small. | Forecasted via a time series regression. | 1. Optimistic scenario: 1st Quarter: 0.0342 2nd Quarter: -0.0033 3rd Quarter: 0.0004 2. Pessimistic scenario: 1st Quarter: 0.0382 2nd Quarter: -0.0013 3rd Quarter: 0.0016 | 1. Forecasts might not reflect the true time path of prices. 2. Large price changes within the microsimulation model could potentially underestimate the welfare effect. |
Remittances | 1. GLSS values taken as reflective of remittances. 2. Changes at the national level taken to reflect changes at the household level. | Adjusted with mean values from the GSS rapid surveys | 1. Inflation adjustment: 32.13% 2. Optimistic scenario: 1st Quarter: -0.0572 2nd Quarter: -0.0286 3rd Quarter: 0.0000 3. Pessimistic scenario: 1st Quarter: -0.0687 2nd Quarter: -0.0343 3rd Quarter: -0.0500 | Likely to understate remittances at the household level if the true level of remittances is under-reported in the survey. If the rate of reduction is smaller than assumed, then the value of remittances will be overstated. |
GLSS7 dataset | 1. Expenditure shares stayed the same over time. | 1. Updates using CPI. 2. Sample weights adjusted to reflect 2020 population estimates. | 1. Inflation adjustment: 32.13% 2. Population growth: 11.09% |
-
Source: Authors’ calculations based on GSS rapid surveys.
Share of workers that experienced a decline in wages, and average decline in sales
Percent of workers with reduced wages (%) | Wave 1 | Wave 2 |
---|---|---|
Agriculture and other industries | 11.7 | 17.5 |
Manufacturing | 14.8 | 7.5 |
Trade | 28 | 12 |
Accommodation/Food | 30.5 | 15 |
Other services | 36.3 | 8.4 |
Average decrease in sales (%) | Scenario 1 | Scenario 2 |
Agriculture and other industries | 0.1265 | 0.1569 |
Utility & gas | 0.0287 | 0.0356 |
Manufacturing | 0.0385 | 0.0458 |
Mining & quarrying | 0.0266 | 0.0330 |
Trade | 0.1133 | 0.1325 |
Accommodation/Food | 0.1300 | 0.1328 |
Other services | 0.1272 | 0.1529 |
Construction | 0.0266 | 0.0330 |
-
Source: Authors’ calculations based on GSS rapid surveys.
References
-
1
Strengthening Multi-Country Evidence of COVID-19 on Poverty and Inequality Impact and the Role of Social ProtectionWorking Paper.
-
2
Socio-Economic Impact of COVID-19 on Ghana’s Economy: Challenges and ProspectsInternational Journal of Social Economics 48:543–556.https://doi.org/10.1108/IJSE-08-2020-0582
-
3
Poverty, Inequality and Stochastic Dominance, Theory and Practice: Illustration with Burkina Faso SurveysCanada: CIRPÉE.
-
4
Prices and Welfare: An Introduction to the Measurement of Well-Being When Prices ChangeCham: Palgrave Pivot.https://doi.org/10.1007/978-3-030-17423-1
-
5
Middle East and North Africa Economic Update, April 2020: How Transparency Can Help the Middle East and North Africa [Serial]World Bank.https://doi.org/10.1016/978-1-4648-1561-4
-
6
COVID-19 Pandemic, a War to be Won: Understanding its Economic Implications for AfricaApplied Health Economics and Health Policy 18:325–328.https://doi.org/10.1007/s40258-020-00580-x
-
7
Gender Differences in Poverty and Household Consumption through the Life-Cycle: A Global PerspectiveWorld Bank.
-
8
Impact of COVID-19 on Poverty and Living Standards in Ghana: A Micro-PerspectiveCogent Economics & Finance 9:1879716.https://doi.org/10.1080/23322039.2021.1879716
-
9
Gender Inequality During the COVID-19 Pandemic: Income, Expenditure, Savings, and Job LossWorld Development 140:105296.https://doi.org/10.1016/j.worlddev.2020.105296
- 10
- 11
-
12
Gender Differences in Experiencing Coronavirus-Triggered Economic Hardship: Evidence from Four Developing CountriesResearch in Social Stratification and Mobility 71:100555.https://doi.org/10.1016/j.rssm.2020.100555
-
13
A Long and Difficult Ascent: Vol. World Economic Outlook65–68, The Great Lockdown: Dissecting the Economic Effects, A Long and Difficult Ascent: Vol. World Economic Outlook, International Monetary Fund, p.
-
14
The Impact of COVID-19 Lockdowns and Expanded Social Assistance on Inequality, Poverty and Mobility in Argentina, Brazil, Colombia and MexicoCEQ Institute, Tulane University.
-
15
The Potential Impact of COVID-19 on GDP and Trade: A Preliminary AssessmentWorld Bank.https://doi.org/10.1596/1813-9450-9211
-
16
COVID-19 Water and Electricity Subsidies in Ghana: How do the Poor Benefit?Scientific African 14:e01038.https://doi.org/10.1016/j.sciaf.2021.e01038
- 17
-
18
COVID-19 in Africa: Socio-Economic Impact, Policy Response and OpportunitiesInternational Journal of Sociology and Social Policy 42:177–200.https://doi.org/10.1108/IJSSP-05-2020-0171
-
19
Labour Market Dynamics in South Africa at the Onset of the COVID-19 PandemicThe South African Journal of Economics. Suid-Afrikaanse Tydskrif Vir Ekonomie 89:44–62.https://doi.org/10.1111/saje.12283
-
20
The Labour Market Impact of COVID-19 Lockdowns (27)The Labour Market Impact of COVID-19 Lockdowns (27).
- 21
- 22
- 23
-
24
Sociodemographic Inequality in Exposure to COVID-19-Induced Economic Hardship in the United KingdomResearch in Social Stratification and Mobility 69:100551.https://doi.org/10.1016/j.rssm.2020.100551
- 25
-
26
The Economic Impact of Covid-19 on Women in Vulnerable Sectors and EconomiesThe Economic Impact of Covid-19 on Women in Vulnerable Sectors and Economies, Information Note.
Article and author information
Author details
Funding
This work was carried out with financial and scientific support from the Partnership for Economic Policy (PEP), with funding from the Government of Canada through the International Development Research Centre (IDRC).
Acknowledgements
The authors are grateful to Luca Tiberti, Marjorie Alain, Arnault Christian Emini and PEP staff for their technical support and guidance, to their discussant and the participants at the 2021 annual PEP conference, and to the anonymous reviewers of earlier versions of the paper and the journal referees. The views presented here are the authors’ and do not represent the views of their institutions, PEP or the funders. The usual caveats apply, and all remaining errors are those of the authors.
Publication history
- Version of Record published: December 31, 2022 (version 1)
Copyright
© 2022, Cooke, Araar, Abrokwah, Acheampong, Abeka, AbrokwahAppiah
This article is distributed under the terms of the Creative Commons Attribution License, which permits unrestricted use and redistribution provided that the original author and source are credited.